class: center, middle, inverse, title-slide #
Data Infrastructure and Import
## Analyzing
scRNA-seq
data with
Bioconductor
for
LCG-EJ-UNAM
March 2020 ###
Leonardo Collado-Torres
### 2020-03-23 --- class: inverse .center[ <a href="https://bioconductor.org/"><img src="https://osca.bioconductor.org/cover.png" style="width: 30%"/></a> <a rel="license" href="http://creativecommons.org/licenses/by-nc-sa/4.0/"><img alt="Creative Commons License" style="border-width:0" src="https://i.creativecommons.org/l/by-nc-sa/4.0/88x31.png" /></a><br />This work is licensed under a <a rel="license" href="http://creativecommons.org/licenses/by-nc-sa/4.0/">Creative Commons Attribution-NonCommercial-ShareAlike 4.0 International License</a>. <a href='https://clustrmaps.com/site/1b5pl' title='Visit tracker'><img src='//clustrmaps.com/map_v2.png?cl=ffffff&w=150&t=n&d=tq5q8216epOrQBSllNIKhXOHUHi-i38brzUURkQEiXw'/></a> ] .footnote[ Download the materials for this course with `usethis::use_course('lcolladotor/osca_LIIGH_UNAM_2020')` or view online at [**lcolladotor.github.io/osca_LIIGH_UNAM_2020**](http://lcolladotor.github.io/osca_LIIGH_UNAM_2020).] <style type="text/css"> /* From https://github.com/yihui/xaringan/issues/147 */ .scroll-output { height: 80%; overflow-y: scroll; } /* https://stackoverflow.com/questions/50919104/horizontally-scrollable-output-on-xaringan-slides */ pre { max-width: 100%; overflow-x: scroll; } /* From https://github.com/yihui/xaringan/wiki/Font-Size */ .tiny{ font-size: 40% } /* From https://github.com/yihui/xaringan/wiki/Title-slide */ .title-slide { background-image: url(https://raw.githubusercontent.com/Bioconductor/OrchestratingSingleCellAnalysis/master/images/Workflow.png); background-size: 33%; background-position: 0% 100% } </style> --- # Slides by Peter Hickey View them [here](https://docs.google.com/presentation/d/1X9qP3wNlnn3BMUQhuZwAo4vCV76c33X_M-UnHxkPZpE/edit#slide=id.g7e63e0fe24_0_50) --- # Code and output .scroll-output[ ```r library('scRNAseq') sce.416b <- LunSpikeInData(which = "416b") ``` ``` ## snapshotDate(): 2019-10-22 ``` ``` ## see ?scRNAseq and browseVignettes('scRNAseq') for documentation ``` ``` ## loading from cache ``` ``` ## see ?scRNAseq and browseVignettes('scRNAseq') for documentation ``` ``` ## loading from cache ``` ``` ## see ?scRNAseq and browseVignettes('scRNAseq') for documentation ``` ``` ## loading from cache ``` ```r # Load the SingleCellExperiment package library('SingleCellExperiment') # Extract the count matrix from the 416b dataset counts.416b <- counts(sce.416b) # Construct a new SCE from the counts matrix sce <- SingleCellExperiment(assays = list(counts = counts.416b)) # Inspect the object we just created sce ``` ``` ## class: SingleCellExperiment ## dim: 46604 192 ## metadata(0): ## assays(1): counts ## rownames(46604): ENSMUSG00000102693 ENSMUSG00000064842 ... ## ENSMUSG00000095742 CBFB-MYH11-mcherry ## rowData names(0): ## colnames(192): SLX-9555.N701_S502.C89V9ANXX.s_1.r_1 ## SLX-9555.N701_S503.C89V9ANXX.s_1.r_1 ... ## SLX-11312.N712_S508.H5H5YBBXX.s_8.r_1 ## SLX-11312.N712_S517.H5H5YBBXX.s_8.r_1 ## colData names(0): ## reducedDimNames(0): ## spikeNames(0): ## altExpNames(0): ``` ```r ## How big is it? pryr::object_size(sce) ``` ``` ## Registered S3 method overwritten by 'pryr': ## method from ## print.bytes Rcpp ``` ``` ## 40.1 MB ``` ```r # Access the counts matrix from the assays slot # WARNING: This will flood RStudio with output! # 1. The general method assay(sce, "counts")[1:6, 1:3] ``` ``` ## SLX-9555.N701_S502.C89V9ANXX.s_1.r_1 ## ENSMUSG00000102693 0 ## ENSMUSG00000064842 0 ## ENSMUSG00000051951 0 ## ENSMUSG00000102851 0 ## ENSMUSG00000103377 0 ## ENSMUSG00000104017 0 ## SLX-9555.N701_S503.C89V9ANXX.s_1.r_1 ## ENSMUSG00000102693 0 ## ENSMUSG00000064842 0 ## ENSMUSG00000051951 0 ## ENSMUSG00000102851 0 ## ENSMUSG00000103377 0 ## ENSMUSG00000104017 0 ## SLX-9555.N701_S504.C89V9ANXX.s_1.r_1 ## ENSMUSG00000102693 0 ## ENSMUSG00000064842 0 ## ENSMUSG00000051951 0 ## ENSMUSG00000102851 0 ## ENSMUSG00000103377 0 ## ENSMUSG00000104017 0 ``` ```r # 2. The special method for the assay named "counts" counts(sce)[1:6, 1:3] ``` ``` ## SLX-9555.N701_S502.C89V9ANXX.s_1.r_1 ## ENSMUSG00000102693 0 ## ENSMUSG00000064842 0 ## ENSMUSG00000051951 0 ## ENSMUSG00000102851 0 ## ENSMUSG00000103377 0 ## ENSMUSG00000104017 0 ## SLX-9555.N701_S503.C89V9ANXX.s_1.r_1 ## ENSMUSG00000102693 0 ## ENSMUSG00000064842 0 ## ENSMUSG00000051951 0 ## ENSMUSG00000102851 0 ## ENSMUSG00000103377 0 ## ENSMUSG00000104017 0 ## SLX-9555.N701_S504.C89V9ANXX.s_1.r_1 ## ENSMUSG00000102693 0 ## ENSMUSG00000064842 0 ## ENSMUSG00000051951 0 ## ENSMUSG00000102851 0 ## ENSMUSG00000103377 0 ## ENSMUSG00000104017 0 ``` ```r sce <- scater::logNormCounts(sce) # Inspect the object we just updated sce ``` ``` ## class: SingleCellExperiment ## dim: 46604 192 ## metadata(0): ## assays(2): counts logcounts ## rownames(46604): ENSMUSG00000102693 ENSMUSG00000064842 ... ## ENSMUSG00000095742 CBFB-MYH11-mcherry ## rowData names(0): ## colnames(192): SLX-9555.N701_S502.C89V9ANXX.s_1.r_1 ## SLX-9555.N701_S503.C89V9ANXX.s_1.r_1 ... ## SLX-11312.N712_S508.H5H5YBBXX.s_8.r_1 ## SLX-11312.N712_S517.H5H5YBBXX.s_8.r_1 ## colData names(0): ## reducedDimNames(0): ## spikeNames(0): ## altExpNames(0): ``` ```r ## How big is it? pryr::object_size(sce) ``` ``` ## 112 MB ``` ```r # 1. The general method assay(sce, "logcounts")[1:6, 1:3] ``` ``` ## SLX-9555.N701_S502.C89V9ANXX.s_1.r_1 ## ENSMUSG00000102693 0 ## ENSMUSG00000064842 0 ## ENSMUSG00000051951 0 ## ENSMUSG00000102851 0 ## ENSMUSG00000103377 0 ## ENSMUSG00000104017 0 ## SLX-9555.N701_S503.C89V9ANXX.s_1.r_1 ## ENSMUSG00000102693 0 ## ENSMUSG00000064842 0 ## ENSMUSG00000051951 0 ## ENSMUSG00000102851 0 ## ENSMUSG00000103377 0 ## ENSMUSG00000104017 0 ## SLX-9555.N701_S504.C89V9ANXX.s_1.r_1 ## ENSMUSG00000102693 0 ## ENSMUSG00000064842 0 ## ENSMUSG00000051951 0 ## ENSMUSG00000102851 0 ## ENSMUSG00000103377 0 ## ENSMUSG00000104017 0 ``` ```r # 2. The special method for the assay named "logcounts" logcounts(sce)[1:6, 1:3] ``` ``` ## SLX-9555.N701_S502.C89V9ANXX.s_1.r_1 ## ENSMUSG00000102693 0 ## ENSMUSG00000064842 0 ## ENSMUSG00000051951 0 ## ENSMUSG00000102851 0 ## ENSMUSG00000103377 0 ## ENSMUSG00000104017 0 ## SLX-9555.N701_S503.C89V9ANXX.s_1.r_1 ## ENSMUSG00000102693 0 ## ENSMUSG00000064842 0 ## ENSMUSG00000051951 0 ## ENSMUSG00000102851 0 ## ENSMUSG00000103377 0 ## ENSMUSG00000104017 0 ## SLX-9555.N701_S504.C89V9ANXX.s_1.r_1 ## ENSMUSG00000102693 0 ## ENSMUSG00000064842 0 ## ENSMUSG00000051951 0 ## ENSMUSG00000102851 0 ## ENSMUSG00000103377 0 ## ENSMUSG00000104017 0 ``` ```r # assign a new entry to assays slot assay(sce, "counts_100") <- assay(sce, "counts") + 100 # List the assays in the object assays(sce) ``` ``` ## List of length 3 ## names(3): counts logcounts counts_100 ``` ```r assayNames(sce) ``` ``` ## [1] "counts" "logcounts" "counts_100" ``` ```r ## How big is it? pryr::object_size(sce) ``` ``` ## 183 MB ``` ```r # Extract the sample metadata from the 416b dataset colData.416b <- colData(sce.416b) # Add some of the sample metadata to our SCE colData(sce) <- colData.416b[, c("phenotype", "block")] # Inspect the object we just updated sce ``` ``` ## class: SingleCellExperiment ## dim: 46604 192 ## metadata(0): ## assays(3): counts logcounts counts_100 ## rownames(46604): ENSMUSG00000102693 ENSMUSG00000064842 ... ## ENSMUSG00000095742 CBFB-MYH11-mcherry ## rowData names(0): ## colnames(192): SLX-9555.N701_S502.C89V9ANXX.s_1.r_1 ## SLX-9555.N701_S503.C89V9ANXX.s_1.r_1 ... ## SLX-11312.N712_S508.H5H5YBBXX.s_8.r_1 ## SLX-11312.N712_S517.H5H5YBBXX.s_8.r_1 ## colData names(2): phenotype block ## reducedDimNames(0): ## spikeNames(0): ## altExpNames(0): ``` ```r # Access the sample metadata from our SCE colData(sce) ``` ``` ## DataFrame with 192 rows and 2 columns ## phenotype ## <character> ## SLX-9555.N701_S502.C89V9ANXX.s_1.r_1 wild type phenotype ## SLX-9555.N701_S503.C89V9ANXX.s_1.r_1 wild type phenotype ## SLX-9555.N701_S504.C89V9ANXX.s_1.r_1 wild type phenotype ## SLX-9555.N701_S505.C89V9ANXX.s_1.r_1 induced CBFB-MYH11 oncogene expression ## SLX-9555.N701_S506.C89V9ANXX.s_1.r_1 induced CBFB-MYH11 oncogene expression ## ... ... ## SLX-11312.N712_S505.H5H5YBBXX.s_8.r_1 induced CBFB-MYH11 oncogene expression ## SLX-11312.N712_S506.H5H5YBBXX.s_8.r_1 induced CBFB-MYH11 oncogene expression ## SLX-11312.N712_S507.H5H5YBBXX.s_8.r_1 induced CBFB-MYH11 oncogene expression ## SLX-11312.N712_S508.H5H5YBBXX.s_8.r_1 induced CBFB-MYH11 oncogene expression ## SLX-11312.N712_S517.H5H5YBBXX.s_8.r_1 wild type phenotype ## block ## <integer> ## SLX-9555.N701_S502.C89V9ANXX.s_1.r_1 20160113 ## SLX-9555.N701_S503.C89V9ANXX.s_1.r_1 20160113 ## SLX-9555.N701_S504.C89V9ANXX.s_1.r_1 20160113 ## SLX-9555.N701_S505.C89V9ANXX.s_1.r_1 20160113 ## SLX-9555.N701_S506.C89V9ANXX.s_1.r_1 20160113 ## ... ... ## SLX-11312.N712_S505.H5H5YBBXX.s_8.r_1 20160325 ## SLX-11312.N712_S506.H5H5YBBXX.s_8.r_1 20160325 ## SLX-11312.N712_S507.H5H5YBBXX.s_8.r_1 20160325 ## SLX-11312.N712_S508.H5H5YBBXX.s_8.r_1 20160325 ## SLX-11312.N712_S517.H5H5YBBXX.s_8.r_1 20160325 ``` ```r # Access a specific column of sample metadata from our SCE table(sce$block) ``` ``` ## ## 20160113 20160325 ## 96 96 ``` ```r # Example of function that adds extra fields to colData sce <- scater::addPerCellQC(sce.416b) # Access the sample metadata from our updated SCE colData(sce) ``` ``` ## DataFrame with 192 rows and 22 columns ## Source Name ## <character> ## SLX-9555.N701_S502.C89V9ANXX.s_1.r_1 SLX-9555.N701_S502.C89V9ANXX.s_1.r_1 ## SLX-9555.N701_S503.C89V9ANXX.s_1.r_1 SLX-9555.N701_S503.C89V9ANXX.s_1.r_1 ## SLX-9555.N701_S504.C89V9ANXX.s_1.r_1 SLX-9555.N701_S504.C89V9ANXX.s_1.r_1 ## SLX-9555.N701_S505.C89V9ANXX.s_1.r_1 SLX-9555.N701_S505.C89V9ANXX.s_1.r_1 ## SLX-9555.N701_S506.C89V9ANXX.s_1.r_1 SLX-9555.N701_S506.C89V9ANXX.s_1.r_1 ## ... ... ## SLX-11312.N712_S505.H5H5YBBXX.s_8.r_1 SLX-11312.N712_S505.H5H5YBBXX.s_8.r_1 ## SLX-11312.N712_S506.H5H5YBBXX.s_8.r_1 SLX-11312.N712_S506.H5H5YBBXX.s_8.r_1 ## SLX-11312.N712_S507.H5H5YBBXX.s_8.r_1 SLX-11312.N712_S507.H5H5YBBXX.s_8.r_1 ## SLX-11312.N712_S508.H5H5YBBXX.s_8.r_1 SLX-11312.N712_S508.H5H5YBBXX.s_8.r_1 ## SLX-11312.N712_S517.H5H5YBBXX.s_8.r_1 SLX-11312.N712_S517.H5H5YBBXX.s_8.r_1 ## cell line cell type ## <character> <character> ## SLX-9555.N701_S502.C89V9ANXX.s_1.r_1 416B embryonic stem cell ## SLX-9555.N701_S503.C89V9ANXX.s_1.r_1 416B embryonic stem cell ## SLX-9555.N701_S504.C89V9ANXX.s_1.r_1 416B embryonic stem cell ## SLX-9555.N701_S505.C89V9ANXX.s_1.r_1 416B embryonic stem cell ## SLX-9555.N701_S506.C89V9ANXX.s_1.r_1 416B embryonic stem cell ## ... ... ... ## SLX-11312.N712_S505.H5H5YBBXX.s_8.r_1 416B embryonic stem cell ## SLX-11312.N712_S506.H5H5YBBXX.s_8.r_1 416B embryonic stem cell ## SLX-11312.N712_S507.H5H5YBBXX.s_8.r_1 416B embryonic stem cell ## SLX-11312.N712_S508.H5H5YBBXX.s_8.r_1 416B embryonic stem cell ## SLX-11312.N712_S517.H5H5YBBXX.s_8.r_1 416B embryonic stem cell ## single cell well quality ## <character> ## SLX-9555.N701_S502.C89V9ANXX.s_1.r_1 OK ## SLX-9555.N701_S503.C89V9ANXX.s_1.r_1 OK ## SLX-9555.N701_S504.C89V9ANXX.s_1.r_1 OK ## SLX-9555.N701_S505.C89V9ANXX.s_1.r_1 OK ## SLX-9555.N701_S506.C89V9ANXX.s_1.r_1 OK ## ... ... ## SLX-11312.N712_S505.H5H5YBBXX.s_8.r_1 OK ## SLX-11312.N712_S506.H5H5YBBXX.s_8.r_1 OK ## SLX-11312.N712_S507.H5H5YBBXX.s_8.r_1 OK ## SLX-11312.N712_S508.H5H5YBBXX.s_8.r_1 OK ## SLX-11312.N712_S517.H5H5YBBXX.s_8.r_1 OK ## genotype ## <character> ## SLX-9555.N701_S502.C89V9ANXX.s_1.r_1 Doxycycline-inducible CBFB-MYH11 oncogene ## SLX-9555.N701_S503.C89V9ANXX.s_1.r_1 Doxycycline-inducible CBFB-MYH11 oncogene ## SLX-9555.N701_S504.C89V9ANXX.s_1.r_1 Doxycycline-inducible CBFB-MYH11 oncogene ## SLX-9555.N701_S505.C89V9ANXX.s_1.r_1 Doxycycline-inducible CBFB-MYH11 oncogene ## SLX-9555.N701_S506.C89V9ANXX.s_1.r_1 Doxycycline-inducible CBFB-MYH11 oncogene ## ... ... ## SLX-11312.N712_S505.H5H5YBBXX.s_8.r_1 Doxycycline-inducible CBFB-MYH11 oncogene ## SLX-11312.N712_S506.H5H5YBBXX.s_8.r_1 Doxycycline-inducible CBFB-MYH11 oncogene ## SLX-11312.N712_S507.H5H5YBBXX.s_8.r_1 Doxycycline-inducible CBFB-MYH11 oncogene ## SLX-11312.N712_S508.H5H5YBBXX.s_8.r_1 Doxycycline-inducible CBFB-MYH11 oncogene ## SLX-11312.N712_S517.H5H5YBBXX.s_8.r_1 Doxycycline-inducible CBFB-MYH11 oncogene ## phenotype ## <character> ## SLX-9555.N701_S502.C89V9ANXX.s_1.r_1 wild type phenotype ## SLX-9555.N701_S503.C89V9ANXX.s_1.r_1 wild type phenotype ## SLX-9555.N701_S504.C89V9ANXX.s_1.r_1 wild type phenotype ## SLX-9555.N701_S505.C89V9ANXX.s_1.r_1 induced CBFB-MYH11 oncogene expression ## SLX-9555.N701_S506.C89V9ANXX.s_1.r_1 induced CBFB-MYH11 oncogene expression ## ... ... ## SLX-11312.N712_S505.H5H5YBBXX.s_8.r_1 induced CBFB-MYH11 oncogene expression ## SLX-11312.N712_S506.H5H5YBBXX.s_8.r_1 induced CBFB-MYH11 oncogene expression ## SLX-11312.N712_S507.H5H5YBBXX.s_8.r_1 induced CBFB-MYH11 oncogene expression ## SLX-11312.N712_S508.H5H5YBBXX.s_8.r_1 induced CBFB-MYH11 oncogene expression ## SLX-11312.N712_S517.H5H5YBBXX.s_8.r_1 wild type phenotype ## strain spike-in addition block ## <character> <character> <integer> ## SLX-9555.N701_S502.C89V9ANXX.s_1.r_1 B6D2F1-J ERCC+SIRV 20160113 ## SLX-9555.N701_S503.C89V9ANXX.s_1.r_1 B6D2F1-J ERCC+SIRV 20160113 ## SLX-9555.N701_S504.C89V9ANXX.s_1.r_1 B6D2F1-J ERCC+SIRV 20160113 ## SLX-9555.N701_S505.C89V9ANXX.s_1.r_1 B6D2F1-J ERCC+SIRV 20160113 ## SLX-9555.N701_S506.C89V9ANXX.s_1.r_1 B6D2F1-J ERCC+SIRV 20160113 ## ... ... ... ... ## SLX-11312.N712_S505.H5H5YBBXX.s_8.r_1 B6D2F1-J Premixed 20160325 ## SLX-11312.N712_S506.H5H5YBBXX.s_8.r_1 B6D2F1-J Premixed 20160325 ## SLX-11312.N712_S507.H5H5YBBXX.s_8.r_1 B6D2F1-J Premixed 20160325 ## SLX-11312.N712_S508.H5H5YBBXX.s_8.r_1 B6D2F1-J Premixed 20160325 ## SLX-11312.N712_S517.H5H5YBBXX.s_8.r_1 B6D2F1-J Premixed 20160325 ## sum detected percent_top_50 ## <integer> <integer> <numeric> ## SLX-9555.N701_S502.C89V9ANXX.s_1.r_1 865936 7618 26.7218362557972 ## SLX-9555.N701_S503.C89V9ANXX.s_1.r_1 1076277 7521 29.4043262097025 ## SLX-9555.N701_S504.C89V9ANXX.s_1.r_1 1180138 8306 27.3453613052033 ## SLX-9555.N701_S505.C89V9ANXX.s_1.r_1 1342593 8143 35.809213961342 ## SLX-9555.N701_S506.C89V9ANXX.s_1.r_1 1668311 7154 34.1197774275899 ## ... ... ... ... ## SLX-11312.N712_S505.H5H5YBBXX.s_8.r_1 776622 8174 45.936246977294 ## SLX-11312.N712_S506.H5H5YBBXX.s_8.r_1 1299950 8956 38.0829262663949 ## SLX-11312.N712_S507.H5H5YBBXX.s_8.r_1 1800696 9530 30.6675307769885 ## SLX-11312.N712_S508.H5H5YBBXX.s_8.r_1 46731 6649 32.2997581904945 ## SLX-11312.N712_S517.H5H5YBBXX.s_8.r_1 1866692 10964 26.6632095707273 ## percent_top_100 percent_top_200 ## <numeric> <numeric> ## SLX-9555.N701_S502.C89V9ANXX.s_1.r_1 32.2773276546997 39.7208338722492 ## SLX-9555.N701_S503.C89V9ANXX.s_1.r_1 35.0354044544295 42.258080401235 ## SLX-9555.N701_S504.C89V9ANXX.s_1.r_1 32.4769645583822 39.3295529844815 ## SLX-9555.N701_S505.C89V9ANXX.s_1.r_1 40.2666332984009 46.2459583805368 ## SLX-9555.N701_S506.C89V9ANXX.s_1.r_1 39.0901336741171 45.6660059185607 ## ... ... ... ## SLX-11312.N712_S505.H5H5YBBXX.s_8.r_1 49.7010128479492 54.6100934560185 ## SLX-11312.N712_S506.H5H5YBBXX.s_8.r_1 42.8929574214393 49.0621946997961 ## SLX-11312.N712_S507.H5H5YBBXX.s_8.r_1 35.5838520216627 41.8550382740896 ## SLX-11312.N712_S508.H5H5YBBXX.s_8.r_1 37.9148744944469 44.5999443624147 ## SLX-11312.N712_S517.H5H5YBBXX.s_8.r_1 31.2583972074665 37.5607759608977 ## percent_top_500 altexps_ERCC_sum ## <numeric> <integer> ## SLX-9555.N701_S502.C89V9ANXX.s_1.r_1 52.9037942757894 65278 ## SLX-9555.N701_S503.C89V9ANXX.s_1.r_1 55.7454075484285 74748 ## SLX-9555.N701_S504.C89V9ANXX.s_1.r_1 51.9336721637639 60878 ## SLX-9555.N701_S505.C89V9ANXX.s_1.r_1 57.1209592184675 60073 ## SLX-9555.N701_S506.C89V9ANXX.s_1.r_1 58.2004194661547 136810 ## ... ... ... ## SLX-11312.N712_S505.H5H5YBBXX.s_8.r_1 64.4249068401359 61575 ## SLX-11312.N712_S506.H5H5YBBXX.s_8.r_1 60.6674872110466 94982 ## SLX-11312.N712_S507.H5H5YBBXX.s_8.r_1 53.6780778099135 113707 ## SLX-11312.N712_S508.H5H5YBBXX.s_8.r_1 56.5235068798014 7580 ## SLX-11312.N712_S517.H5H5YBBXX.s_8.r_1 48.9489428357758 48664 ## altexps_ERCC_detected ## <integer> ## SLX-9555.N701_S502.C89V9ANXX.s_1.r_1 39 ## SLX-9555.N701_S503.C89V9ANXX.s_1.r_1 40 ## SLX-9555.N701_S504.C89V9ANXX.s_1.r_1 42 ## SLX-9555.N701_S505.C89V9ANXX.s_1.r_1 42 ## SLX-9555.N701_S506.C89V9ANXX.s_1.r_1 44 ## ... ... ## SLX-11312.N712_S505.H5H5YBBXX.s_8.r_1 39 ## SLX-11312.N712_S506.H5H5YBBXX.s_8.r_1 41 ## SLX-11312.N712_S507.H5H5YBBXX.s_8.r_1 40 ## SLX-11312.N712_S508.H5H5YBBXX.s_8.r_1 44 ## SLX-11312.N712_S517.H5H5YBBXX.s_8.r_1 39 ## altexps_ERCC_percent altexps_SIRV_sum ## <numeric> <integer> ## SLX-9555.N701_S502.C89V9ANXX.s_1.r_1 6.80658407035354 27828 ## SLX-9555.N701_S503.C89V9ANXX.s_1.r_1 6.28029958040595 39173 ## SLX-9555.N701_S504.C89V9ANXX.s_1.r_1 4.78949297995239 30058 ## SLX-9555.N701_S505.C89V9ANXX.s_1.r_1 4.18566507433069 32542 ## SLX-9555.N701_S506.C89V9ANXX.s_1.r_1 7.28887127185236 71850 ## ... ... ... ## SLX-11312.N712_S505.H5H5YBBXX.s_8.r_1 7.17619705260214 19848 ## SLX-11312.N712_S506.H5H5YBBXX.s_8.r_1 6.65764326634008 31729 ## SLX-11312.N712_S507.H5H5YBBXX.s_8.r_1 5.81467119470586 41116 ## SLX-11312.N712_S508.H5H5YBBXX.s_8.r_1 13.488984589102 1883 ## SLX-11312.N712_S517.H5H5YBBXX.s_8.r_1 2.51930349520745 16289 ## altexps_SIRV_detected ## <integer> ## SLX-9555.N701_S502.C89V9ANXX.s_1.r_1 7 ## SLX-9555.N701_S503.C89V9ANXX.s_1.r_1 7 ## SLX-9555.N701_S504.C89V9ANXX.s_1.r_1 7 ## SLX-9555.N701_S505.C89V9ANXX.s_1.r_1 7 ## SLX-9555.N701_S506.C89V9ANXX.s_1.r_1 7 ## ... ... ## SLX-11312.N712_S505.H5H5YBBXX.s_8.r_1 7 ## SLX-11312.N712_S506.H5H5YBBXX.s_8.r_1 7 ## SLX-11312.N712_S507.H5H5YBBXX.s_8.r_1 7 ## SLX-11312.N712_S508.H5H5YBBXX.s_8.r_1 7 ## SLX-11312.N712_S517.H5H5YBBXX.s_8.r_1 7 ## altexps_SIRV_percent total ## <numeric> <integer> ## SLX-9555.N701_S502.C89V9ANXX.s_1.r_1 2.9016456005055 959042 ## SLX-9555.N701_S503.C89V9ANXX.s_1.r_1 3.29130111124368 1190198 ## SLX-9555.N701_S504.C89V9ANXX.s_1.r_1 2.36477183861837 1271074 ## SLX-9555.N701_S505.C89V9ANXX.s_1.r_1 2.26740653619545 1435208 ## SLX-9555.N701_S506.C89V9ANXX.s_1.r_1 3.82797603159559 1876971 ## ... ... ... ## SLX-11312.N712_S505.H5H5YBBXX.s_8.r_1 2.31316539342342 858045 ## SLX-11312.N712_S506.H5H5YBBXX.s_8.r_1 2.22400416076419 1426661 ## SLX-11312.N712_S507.H5H5YBBXX.s_8.r_1 2.10256203084705 1955519 ## SLX-11312.N712_S508.H5H5YBBXX.s_8.r_1 3.35089155425846 56194 ## SLX-11312.N712_S517.H5H5YBBXX.s_8.r_1 0.843270890872805 1931645 ``` ```r # Inspect the object we just updated sce ``` ``` ## class: SingleCellExperiment ## dim: 46604 192 ## metadata(0): ## assays(1): counts ## rownames(46604): ENSMUSG00000102693 ENSMUSG00000064842 ... ## ENSMUSG00000095742 CBFB-MYH11-mcherry ## rowData names(1): Length ## colnames(192): SLX-9555.N701_S502.C89V9ANXX.s_1.r_1 ## SLX-9555.N701_S503.C89V9ANXX.s_1.r_1 ... ## SLX-11312.N712_S508.H5H5YBBXX.s_8.r_1 ## SLX-11312.N712_S517.H5H5YBBXX.s_8.r_1 ## colData names(22): Source Name cell line ... altexps_SIRV_percent total ## reducedDimNames(0): ## spikeNames(0): ## altExpNames(2): ERCC SIRV ``` ```r ## How big is it? pryr::object_size(sce) ``` ``` ## 40.8 MB ``` ```r ## Add the lognorm counts again sce <- scater::logNormCounts(sce) ## How big is it? pryr::object_size(sce) ``` ``` ## 112 MB ``` ```r # E.g., subset data to just wild type cells # Remember, cells are columns of the SCE sce[, sce$phenotype == "wild type phenotype"] ``` ``` ## class: SingleCellExperiment ## dim: 46604 96 ## metadata(0): ## assays(2): counts logcounts ## rownames(46604): ENSMUSG00000102693 ENSMUSG00000064842 ... ## ENSMUSG00000095742 CBFB-MYH11-mcherry ## rowData names(1): Length ## colnames(96): SLX-9555.N701_S502.C89V9ANXX.s_1.r_1 ## SLX-9555.N701_S503.C89V9ANXX.s_1.r_1 ... ## SLX-11312.N712_S504.H5H5YBBXX.s_8.r_1 ## SLX-11312.N712_S517.H5H5YBBXX.s_8.r_1 ## colData names(22): Source Name cell line ... altexps_SIRV_percent total ## reducedDimNames(0): ## spikeNames(0): ## altExpNames(2): ERCC SIRV ``` ```r # Access the feature metadata from our SCE # It's currently empty! rowData(sce) ``` ``` ## DataFrame with 46604 rows and 1 column ## Length ## <integer> ## ENSMUSG00000102693 1070 ## ENSMUSG00000064842 110 ## ENSMUSG00000051951 6094 ## ENSMUSG00000102851 480 ## ENSMUSG00000103377 2819 ## ... ... ## ENSMUSG00000094621 121 ## ENSMUSG00000098647 99 ## ENSMUSG00000096730 3077 ## ENSMUSG00000095742 243 ## CBFB-MYH11-mcherry 2998 ``` ```r # Example of function that adds extra fields to rowData sce <- scater::addPerFeatureQC(sce) # Access the feature metadata from our updated SCE rowData(sce) ``` ``` ## DataFrame with 46604 rows and 3 columns ## Length mean detected ## <integer> <numeric> <numeric> ## ENSMUSG00000102693 1070 0 0 ## ENSMUSG00000064842 110 0 0 ## ENSMUSG00000051951 6094 0 0 ## ENSMUSG00000102851 480 0 0 ## ENSMUSG00000103377 2819 0.0104166666666667 0.520833333333333 ## ... ... ... ... ## ENSMUSG00000094621 121 0 0 ## ENSMUSG00000098647 99 0 0 ## ENSMUSG00000096730 3077 0 0 ## ENSMUSG00000095742 243 0 0 ## CBFB-MYH11-mcherry 2998 50375.7135416667 100 ``` ```r ## How big is it? pryr::object_size(sce) ``` ``` ## 113 MB ``` ```r # Download the relevant Ensembl annotation database # using AnnotationHub resources library('AnnotationHub') ah <- AnnotationHub() ``` ``` ## snapshotDate(): 2019-10-29 ``` ```r query(ah, c("Mus musculus", "Ensembl", "v97")) ``` ``` ## AnnotationHub with 1 record ## # snapshotDate(): 2019-10-29 ## # names(): AH73905 ## # $dataprovider: Ensembl ## # $species: Mus musculus ## # $rdataclass: EnsDb ## # $rdatadateadded: 2019-05-02 ## # $title: Ensembl 97 EnsDb for Mus musculus ## # $description: Gene and protein annotations for Mus musculus based on Ensem... ## # $taxonomyid: 10090 ## # $genome: GRCm38 ## # $sourcetype: ensembl ## # $sourceurl: http://www.ensembl.org ## # $sourcesize: NA ## # $tags: c("97", "AHEnsDbs", "Annotation", "EnsDb", "Ensembl", "Gene", ## # "Protein", "Transcript") ## # retrieve record with 'object[["AH73905"]]' ``` ```r # Annotate each gene with its chromosome location ensdb <- ah[["AH73905"]] ``` ``` ## loading from cache ``` ```r chromosome <- mapIds(ensdb, keys = rownames(sce), keytype = "GENEID", column = "SEQNAME") ``` ``` ## Warning: Unable to map 563 of 46604 requested IDs. ``` ```r rowData(sce)$chromosome <- chromosome # Access the feature metadata from our updated SCE rowData(sce) ``` ``` ## DataFrame with 46604 rows and 4 columns ## Length mean detected chromosome ## <integer> <numeric> <numeric> <character> ## ENSMUSG00000102693 1070 0 0 1 ## ENSMUSG00000064842 110 0 0 1 ## ENSMUSG00000051951 6094 0 0 1 ## ENSMUSG00000102851 480 0 0 1 ## ENSMUSG00000103377 2819 0.0104166666666667 0.520833333333333 1 ## ... ... ... ... ... ## ENSMUSG00000094621 121 0 0 GL456372.1 ## ENSMUSG00000098647 99 0 0 GL456381.1 ## ENSMUSG00000096730 3077 0 0 JH584292.1 ## ENSMUSG00000095742 243 0 0 JH584295.1 ## CBFB-MYH11-mcherry 2998 50375.7135416667 100 NA ``` ```r ## How big is it? pryr::object_size(sce) ``` ``` ## 113 MB ``` ```r # E.g., subset data to just genes on chromosome 3 # NOTE: which() needed to cope with NA chromosome names sce[which(rowData(sce)$chromosome == "3"), ] ``` ``` ## class: SingleCellExperiment ## dim: 2876 192 ## metadata(0): ## assays(2): counts logcounts ## rownames(2876): ENSMUSG00000098982 ENSMUSG00000098307 ... ## ENSMUSG00000105990 ENSMUSG00000075903 ## rowData names(4): Length mean detected chromosome ## colnames(192): SLX-9555.N701_S502.C89V9ANXX.s_1.r_1 ## SLX-9555.N701_S503.C89V9ANXX.s_1.r_1 ... ## SLX-11312.N712_S508.H5H5YBBXX.s_8.r_1 ## SLX-11312.N712_S517.H5H5YBBXX.s_8.r_1 ## colData names(22): Source Name cell line ... altexps_SIRV_percent total ## reducedDimNames(0): ## spikeNames(0): ## altExpNames(2): ERCC SIRV ``` ```r # Access the metadata from our SCE # It's currently empty! metadata(sce) ``` ``` ## list() ``` ```r # The metadata slot is Vegas - anything goes metadata(sce) <- list(favourite_genes = c("Shh", "Nck1", "Diablo"), analyst = c("Pete")) # Access the metadata from our updated SCE metadata(sce) ``` ``` ## $favourite_genes ## [1] "Shh" "Nck1" "Diablo" ## ## $analyst ## [1] "Pete" ``` ```r # E.g., add the PCA of logcounts # NOTE: We'll learn more about PCA later sce <- scater::runPCA(sce) # Inspect the object we just updated sce ``` ``` ## class: SingleCellExperiment ## dim: 46604 192 ## metadata(2): favourite_genes analyst ## assays(2): counts logcounts ## rownames(46604): ENSMUSG00000102693 ENSMUSG00000064842 ... ## ENSMUSG00000095742 CBFB-MYH11-mcherry ## rowData names(4): Length mean detected chromosome ## colnames(192): SLX-9555.N701_S502.C89V9ANXX.s_1.r_1 ## SLX-9555.N701_S503.C89V9ANXX.s_1.r_1 ... ## SLX-11312.N712_S508.H5H5YBBXX.s_8.r_1 ## SLX-11312.N712_S517.H5H5YBBXX.s_8.r_1 ## colData names(22): Source Name cell line ... altexps_SIRV_percent total ## reducedDimNames(1): PCA ## spikeNames(0): ## altExpNames(2): ERCC SIRV ``` ```r # Access the PCA matrix from the reducedDims slot reducedDim(sce, "PCA")[1:6, 1:3] ``` ``` ## PC1 PC2 PC3 ## SLX-9555.N701_S502.C89V9ANXX.s_1.r_1 18.717668 -27.598132 5.939654 ## SLX-9555.N701_S503.C89V9ANXX.s_1.r_1 2.480705 -27.564583 4.916567 ## SLX-9555.N701_S504.C89V9ANXX.s_1.r_1 42.034018 -7.552435 12.126964 ## SLX-9555.N701_S505.C89V9ANXX.s_1.r_1 -8.494303 31.833727 15.760853 ## SLX-9555.N701_S506.C89V9ANXX.s_1.r_1 -49.737390 4.226795 6.123169 ## SLX-9555.N701_S507.C89V9ANXX.s_1.r_1 -44.528081 -3.215503 10.384939 ``` ```r # E.g., add a t-SNE representation of logcounts # NOTE: We'll learn more about t-SNE later sce <- scater::runTSNE(sce) # Inspect the object we just updated sce ``` ``` ## class: SingleCellExperiment ## dim: 46604 192 ## metadata(2): favourite_genes analyst ## assays(2): counts logcounts ## rownames(46604): ENSMUSG00000102693 ENSMUSG00000064842 ... ## ENSMUSG00000095742 CBFB-MYH11-mcherry ## rowData names(4): Length mean detected chromosome ## colnames(192): SLX-9555.N701_S502.C89V9ANXX.s_1.r_1 ## SLX-9555.N701_S503.C89V9ANXX.s_1.r_1 ... ## SLX-11312.N712_S508.H5H5YBBXX.s_8.r_1 ## SLX-11312.N712_S517.H5H5YBBXX.s_8.r_1 ## colData names(22): Source Name cell line ... altexps_SIRV_percent total ## reducedDimNames(2): PCA TSNE ## spikeNames(0): ## altExpNames(2): ERCC SIRV ``` ```r # Access the t-SNE matrix from the reducedDims slot head(reducedDim(sce, "TSNE")) ``` ``` ## [,1] [,2] ## SLX-9555.N701_S502.C89V9ANXX.s_1.r_1 2.969316 3.326793 ## SLX-9555.N701_S503.C89V9ANXX.s_1.r_1 4.156261 -0.242203 ## SLX-9555.N701_S504.C89V9ANXX.s_1.r_1 3.223373 5.769899 ## SLX-9555.N701_S505.C89V9ANXX.s_1.r_1 -1.112877 4.476084 ## SLX-9555.N701_S506.C89V9ANXX.s_1.r_1 -6.867466 -7.727206 ## SLX-9555.N701_S507.C89V9ANXX.s_1.r_1 -3.495641 -6.068512 ``` ```r # E.g., add a 'manual' UMAP representation of logcounts # NOTE: We'll learn more about UMAP later and a # simpler way to compute it. u <- uwot::umap(t(logcounts(sce)), n_components = 2) # Add the UMAP matrix to the reducedDims slot # Access the UMAP matrix from the reducedDims slot reducedDim(sce, "UMAP") <- u # List the dimensionality reduction results stored in # the object reducedDims(sce) ``` ``` ## List of length 3 ## names(3): PCA TSNE UMAP ``` ```r # Extract the ERCC SCE from the 416b dataset ercc.sce.416b <- altExp(sce.416b, "ERCC") # Inspect the ERCC SCE ercc.sce.416b ``` ``` ## class: SingleCellExperiment ## dim: 92 192 ## metadata(0): ## assays(1): counts ## rownames(92): ERCC-00002 ERCC-00003 ... ERCC-00170 ERCC-00171 ## rowData names(1): Length ## colnames(192): SLX-9555.N701_S502.C89V9ANXX.s_1.r_1 ## SLX-9555.N701_S503.C89V9ANXX.s_1.r_1 ... ## SLX-11312.N712_S508.H5H5YBBXX.s_8.r_1 ## SLX-11312.N712_S517.H5H5YBBXX.s_8.r_1 ## colData names(0): ## reducedDimNames(0): ## spikeNames(0): ## altExpNames(0): ``` ```r # Add the ERCC SCE as an alternative experiment to our SCE altExp(sce, "ERCC") <- ercc.sce.416b # Inspect the object we just updated sce ``` ``` ## class: SingleCellExperiment ## dim: 46604 192 ## metadata(2): favourite_genes analyst ## assays(2): counts logcounts ## rownames(46604): ENSMUSG00000102693 ENSMUSG00000064842 ... ## ENSMUSG00000095742 CBFB-MYH11-mcherry ## rowData names(4): Length mean detected chromosome ## colnames(192): SLX-9555.N701_S502.C89V9ANXX.s_1.r_1 ## SLX-9555.N701_S503.C89V9ANXX.s_1.r_1 ... ## SLX-11312.N712_S508.H5H5YBBXX.s_8.r_1 ## SLX-11312.N712_S517.H5H5YBBXX.s_8.r_1 ## colData names(22): Source Name cell line ... altexps_SIRV_percent total ## reducedDimNames(3): PCA TSNE UMAP ## spikeNames(0): ## altExpNames(2): ERCC SIRV ``` ```r ## How big is it? pryr::object_size(sce) ``` ``` ## 113 MB ``` ```r # List the alternative experiments stored in the object altExps(sce) ``` ``` ## List of length 2 ## names(2): ERCC SIRV ``` ```r # Subsetting the SCE by sample also subsets the # alternative experiments sce.subset <- sce[, 1:10] ncol(sce.subset) ``` ``` ## [1] 10 ``` ```r ncol(altExp(sce.subset)) ``` ``` ## [1] 10 ``` ```r ## How big is it? pryr::object_size(sce.subset) ``` ``` ## 12 MB ``` ```r # Extract existing size factors (these were added # when we ran scater::logNormCounts(sce)) head(sizeFactors(sce)) ``` ``` ## SLX-9555.N701_S502.C89V9ANXX.s_1.r_1 SLX-9555.N701_S503.C89V9ANXX.s_1.r_1 ## 0.7427411 0.9231573 ## SLX-9555.N701_S504.C89V9ANXX.s_1.r_1 SLX-9555.N701_S505.C89V9ANXX.s_1.r_1 ## 1.0122422 1.1515851 ## SLX-9555.N701_S506.C89V9ANXX.s_1.r_1 SLX-9555.N701_S507.C89V9ANXX.s_1.r_1 ## 1.4309639 0.8713409 ``` ```r # 'Automatically' replace size factors sce <- scran::computeSumFactors(sce) head(sizeFactors(sce)) ``` ``` ## [1] 0.6961756 0.8834223 0.9704247 0.9804890 1.2446699 0.7922620 ``` ```r # 'Manually' replace size factors sizeFactors(sce) <- scater::librarySizeFactors(sce) head(sizeFactors(sce)) ``` ``` ## SLX-9555.N701_S502.C89V9ANXX.s_1.r_1 SLX-9555.N701_S503.C89V9ANXX.s_1.r_1 ## 0.7427411 0.9231573 ## SLX-9555.N701_S504.C89V9ANXX.s_1.r_1 SLX-9555.N701_S505.C89V9ANXX.s_1.r_1 ## 1.0122422 1.1515851 ## SLX-9555.N701_S506.C89V9ANXX.s_1.r_1 SLX-9555.N701_S507.C89V9ANXX.s_1.r_1 ## 1.4309639 0.8713409 ``` ] --- # `sce` object exercises -- * Which function defines the `sce` class? -- * What are the minimum type of tables an `sce` object contains? -- * Where are the `colnames(sce)` used? -- * Similarly, where are the `rownames(sce)` used? -- * How many principal components did we compute? -- * Which three chromosomes have the highest mean gene expression? ??? * `SingleCellExperiment::SingleCellExperiment` * `colData()`, `assays()` and `rowData()` with `reducedDims()` being optional * `rownames(colData())` and `colnames(assays())` * `rownames(rowData())` and `rownames(assays())` * `ncol(reducedDim(sce, 'PCA'))` * `sort(with(rowData(sce), tapply(mean, chromosome, mean)), decreasing = TRUE)` --- # ERCC exercise -- * Make a plot for each sample showing the ERCC counts against the expected. -- * [ERCC expected counts file](https://tools.thermofisher.com/content/sfs/manuals/cms_095046.txt) --- # ERCC solution ```r ## Read the data from the web ercc_info <- read.delim( 'https://tools.thermofisher.com/content/sfs/manuals/cms_095046.txt', as.is = TRUE, row.names = 2, check.names = FALSE ) ## Match the ERCC data m <- match(rownames(altExp(sce, "ERCC")), rownames(ercc_info)) ercc_info <- ercc_info[m, ] ## Normalize the ERCC counts altExp(sce, "ERCC") <- scater::logNormCounts(altExp(sce, "ERCC")) ``` --- .scroll-output[ ```r for (i in seq_len(2)) { plot( log2(10 * ercc_info[, "concentration in Mix 1 (attomoles/ul)"] + 1) ~ log2(counts(altExp(sce, "ERCC"))[, i] + 1), xlab = "log norm counts", ylab = "Mix 1: log2(10 * Concentration + 1)", main = colnames(altExp(sce, "ERCC"))[i], xlim = c(min(logcounts( altExp(sce, "ERCC") )), max(logcounts( altExp(sce, "ERCC") ))) ) abline(0, 1, lty = 2, col = 'red') } ``` 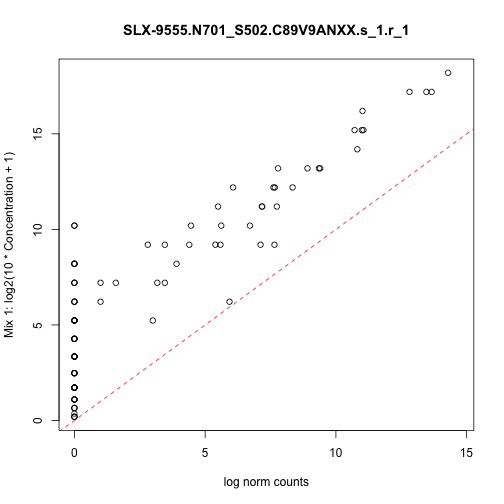<!-- -->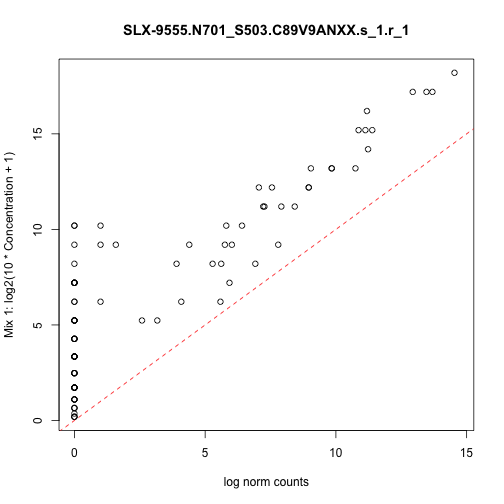<!-- --> ] --- # Importing data .scroll-output[ ```r # Download example data processed with CellRanger # Aside: Using BiocFileCache means we only download the # data once library('BiocFileCache') bfc <- BiocFileCache() pbmc.url <- paste0( "http://cf.10xgenomics.com/samples/cell-vdj/", "3.1.0/vdj_v1_hs_pbmc3/", "vdj_v1_hs_pbmc3_filtered_feature_bc_matrix.tar.gz" ) pbmc.data <- bfcrpath(bfc, pbmc.url) # Extract the files to a temporary location untar(pbmc.data, exdir = tempdir()) # List the files we downloaded and extracted # These files are typically CellRanger outputs pbmc.dir <- file.path(tempdir(), "filtered_feature_bc_matrix") list.files(pbmc.dir) ``` ``` ## [1] "barcodes.tsv.gz" "features.tsv.gz" "matrix.mtx.gz" ``` ```r # Import the data as a SingleCellExperiment library('DropletUtils') sce.pbmc <- read10xCounts(pbmc.dir) # Inspect the object we just constructed sce.pbmc ``` ``` ## class: SingleCellExperiment ## dim: 33555 7231 ## metadata(1): Samples ## assays(1): counts ## rownames(33555): ENSG00000243485 ENSG00000237613 ... CD127 CD15 ## rowData names(3): ID Symbol Type ## colnames: NULL ## colData names(2): Sample Barcode ## reducedDimNames(0): ## spikeNames(0): ## altExpNames(0): ``` ```r ## How big is it? pryr::object_size(sce.pbmc) ``` ``` ## 130 MB ``` ```r # Store the CITE-seq data in an alternative experiment sce.pbmc <- splitAltExps(sce.pbmc, rowData(sce.pbmc)$Type) # Inspect the object we just updated sce.pbmc ``` ``` ## class: SingleCellExperiment ## dim: 33538 7231 ## metadata(1): Samples ## assays(1): counts ## rownames(33538): ENSG00000243485 ENSG00000237613 ... ENSG00000277475 ## ENSG00000268674 ## rowData names(3): ID Symbol Type ## colnames: NULL ## colData names(2): Sample Barcode ## reducedDimNames(0): ## spikeNames(0): ## altExpNames(1): Antibody Capture ``` ```r ## How big is it? pryr::object_size(sce.pbmc) ``` ``` ## 131 MB ``` ```r # Download example data processed with scPipe library('BiocFileCache') bfc <- BiocFileCache() sis_seq.url <- "https://github.com/LuyiTian/SIS-seq_script/archive/master.zip" sis_seq.data <- bfcrpath(bfc, sis_seq.url) # Extract the files to a temporary location unzip(sis_seq.data, exdir = tempdir()) # List (some of) the files we downloaded and extracted # These files are typical scPipe outputs sis_seq.dir <- file.path(tempdir(), "SIS-seq_script-master", "data", "BcorKO_scRNAseq", "RPI10") list.files(sis_seq.dir) ``` ``` ## [1] "gene_count.csv" "stat" ``` ```r # Import the data as a SingleCellExperiment library('scPipe') sce.sis_seq <- create_sce_by_dir(sis_seq.dir) ``` ``` ## organism/gene_id_type not provided. Make a guess: mmusculus_gene_ensembl / ensembl_gene_id ``` ```r # Inspect the object we just constructed sce.sis_seq ``` ``` ## class: SingleCellExperiment ## dim: 19232 383 ## metadata(2): scPipe Biomart ## assays(1): counts ## rownames(19232): ENSMUSG00000079140 ENSMUSG00000081587 ... ## ENSMUSG00000036880 ENSMUSG00000106872 ## rowData names(0): ## colnames(383): A1 A10 ... P8 P9 ## colData names(7): unaligned aligned_unmapped ... mapped_to_ERCC ## mapped_to_MT ## reducedDimNames(0): ## spikeNames(0): ## altExpNames(0): ``` ```r ## How big is it? pryr::object_size(sce.sis_seq) ``` ``` ## 31.3 MB ``` ```r # Download example bunch o' files dataset library('BiocFileCache') bfc <- BiocFileCache() lun_counts.url <- paste0( "https://www.ebi.ac.uk/arrayexpress/files/", "E-MTAB-5522/E-MTAB-5522.processed.1.zip" ) lun_counts.data <- bfcrpath(bfc, lun_counts.url) lun_coldata.url <- paste0("https://www.ebi.ac.uk/arrayexpress/files/", "E-MTAB-5522/E-MTAB-5522.sdrf.txt") lun_coldata.data <- bfcrpath(bfc, lun_coldata.url) # Extract the counts files to a temporary location lun_counts.dir <- tempfile("lun_counts.") unzip(lun_counts.data, exdir = lun_counts.dir) # List the files we downloaded and extracted list.files(lun_counts.dir) ``` ``` ## [1] "counts_Calero_20160113.tsv" "counts_Calero_20160325.tsv" ## [3] "counts_Liora_20160906.tsv" "counts_Liora_20170201.tsv" ``` ```r # Import the count matrix (for 1 plate) lun.counts <- read.delim( file.path(lun_counts.dir, "counts_Calero_20160113.tsv"), header = TRUE, row.names = 1, check.names = FALSE ) # Store the gene lengths for later gene.lengths <- lun.counts$Length # Convert the gene counts to a matrix lun.counts <- as.matrix(lun.counts[, -1]) # Import the sample metadata lun.coldata <- read.delim(lun_coldata.data, check.names = FALSE, stringsAsFactors = FALSE) library('S4Vectors') lun.coldata <- as(lun.coldata, "DataFrame") # Match up the sample metadata to the counts matrix m <- match(colnames(lun.counts), lun.coldata$`Source Name`) lun.coldata <- lun.coldata[m,] # Construct the feature metadata lun.rowdata <- DataFrame(Length = gene.lengths) # Construct the SingleCellExperiment lun.sce <- SingleCellExperiment( assays = list(assays = lun.counts), colData = lun.coldata, rowData = lun.rowdata ) # Inspect the object we just constructed lun.sce ``` ``` ## class: SingleCellExperiment ## dim: 46703 96 ## metadata(0): ## assays(1): assays ## rownames(46703): ENSMUSG00000102693 ENSMUSG00000064842 ... SIRV7 ## CBFB-MYH11-mcherry ## rowData names(1): Length ## colnames(96): SLX-9555.N701_S502.C89V9ANXX.s_1.r_1 ## SLX-9555.N701_S503.C89V9ANXX.s_1.r_1 ... ## SLX-9555.N712_S508.C89V9ANXX.s_1.r_1 ## SLX-9555.N712_S517.C89V9ANXX.s_1.r_1 ## colData names(50): Source Name Comment[ENA_SAMPLE] ... Factor ## Value[phenotype] Factor Value[block] ## reducedDimNames(0): ## spikeNames(0): ## altExpNames(0): ``` ```r ## How big is it? pryr::object_size(lun.sce) ``` ``` ## 22.5 MB ``` ] --- class: middle .center[ # Thanks! Slides created via the R package [**xaringan**](https://github.com/yihui/xaringan) and themed with [**xaringanthemer**](https://github.com/gadenbuie/xaringanthemer). This course is based on the book [**Orchestrating Single Cell Analysis with Bioconductor**](https://osca.bioconductor.org/) by [Aaron Lun](https://www.linkedin.com/in/aaron-lun-869b5894/), [Robert Amezquita](https://robertamezquita.github.io/), [Stephanie Hicks](https://www.stephaniehicks.com/) and [Raphael Gottardo](http://rglab.org), plus [**WEHI's scRNA-seq course**](https://drive.google.com/drive/folders/1cn5d-Ey7-kkMiex8-74qxvxtCQT6o72h) by [Peter Hickey](https://www.peterhickey.org/). You can find the files for this course at [lcolladotor/osca_LIIGH_UNAM_2020](https://github.com/lcolladotor/osca_LIIGH_UNAM_2020). Instructor: [**Leonardo Collado-Torres**](http://lcolladotor.github.io/). <a href="https://www.libd.org"><img src="img/LIBD_logo.jpg" style="width: 20%" /></a> ] .footnote[ Download the materials for this course with `usethis::use_course('lcolladotor/osca_LIIGH_UNAM_2020')` or view online at [**lcolladotor.github.io/osca_LIIGH_UNAM_2020**](http://lcolladotor.github.io/osca_LIIGH_UNAM_2020).] --- # R session information .scroll-output[ .tiny[ ```r options(width = 120) sessioninfo::session_info() ``` ``` ## ─ Session info ─────────────────────────────────────────────────────────────────────────────────────────────────────── ## setting value ## version R version 3.6.3 (2020-02-29) ## os macOS Catalina 10.15.3 ## system x86_64, darwin15.6.0 ## ui X11 ## language (EN) ## collate en_US.UTF-8 ## ctype en_US.UTF-8 ## tz America/New_York ## date 2020-03-23 ## ## ─ Packages ─────────────────────────────────────────────────────────────────────────────────────────────────────────── ## package * version date lib source ## AnnotationDbi * 1.48.0 2019-10-29 [1] Bioconductor ## AnnotationFilter * 1.10.0 2019-10-29 [1] Bioconductor ## AnnotationHub * 2.18.0 2019-10-29 [1] Bioconductor ## askpass 1.1 2019-01-13 [1] CRAN (R 3.6.0) ## assertthat 0.2.1 2019-03-21 [1] CRAN (R 3.6.0) ## Biobase * 2.46.0 2019-10-29 [1] Bioconductor ## BiocFileCache * 1.10.2 2019-11-08 [1] Bioconductor ## BiocGenerics * 0.32.0 2019-10-29 [1] Bioconductor ## BiocManager 1.30.10 2019-11-16 [1] CRAN (R 3.6.1) ## BiocParallel * 1.20.1 2019-12-21 [1] Bioconductor ## BiocVersion 3.10.1 2019-06-06 [1] Bioconductor ## biomaRt 2.42.0 2019-10-29 [1] Bioconductor ## Biostrings 2.54.0 2019-10-29 [1] Bioconductor ## bit 1.1-15.2 2020-02-10 [1] CRAN (R 3.6.0) ## bit64 0.9-7 2017-05-08 [1] CRAN (R 3.6.0) ## bitops 1.0-6 2013-08-17 [1] CRAN (R 3.6.0) ## blob 1.2.1 2020-01-20 [1] CRAN (R 3.6.0) ## cli 2.0.2 2020-02-28 [1] CRAN (R 3.6.0) ## colorout * 1.2-1 2019-05-07 [1] Github (jalvesaq/colorout@7ea9440) ## colorspace 1.4-1 2019-03-18 [1] CRAN (R 3.6.0) ## crayon 1.3.4 2017-09-16 [1] CRAN (R 3.6.0) ## curl 4.3 2019-12-02 [1] CRAN (R 3.6.0) ## DBI 1.1.0 2019-12-15 [1] CRAN (R 3.6.0) ## dbplyr * 1.4.2 2019-06-17 [1] CRAN (R 3.6.0) ## DelayedArray * 0.12.2 2020-01-06 [1] Bioconductor ## DEoptimR 1.0-8 2016-11-19 [1] CRAN (R 3.6.0) ## digest 0.6.25 2020-02-23 [1] CRAN (R 3.6.0) ## dplyr 0.8.5 2020-03-07 [1] CRAN (R 3.6.0) ## dqrng 0.2.1 2019-05-17 [1] CRAN (R 3.6.0) ## DropletUtils * 1.6.1 2019-10-30 [1] Bioconductor ## edgeR 3.28.1 2020-02-26 [1] Bioconductor ## ensembldb * 2.10.2 2019-11-20 [1] Bioconductor ## evaluate 0.14 2019-05-28 [1] CRAN (R 3.6.0) ## ExperimentHub 1.12.0 2019-10-29 [1] Bioconductor ## fansi 0.4.1 2020-01-08 [1] CRAN (R 3.6.0) ## fastmap 1.0.1 2019-10-08 [1] CRAN (R 3.6.0) ## GenomeInfoDb * 1.22.0 2019-10-29 [1] Bioconductor ## GenomeInfoDbData 1.2.2 2019-10-31 [1] Bioconductor ## GenomicAlignments 1.22.1 2019-11-12 [1] Bioconductor ## GenomicFeatures * 1.38.2 2020-02-15 [1] Bioconductor ## GenomicRanges * 1.38.0 2019-10-29 [1] Bioconductor ## GGally 1.4.0 2018-05-17 [1] CRAN (R 3.6.0) ## ggplot2 * 3.3.0 2020-03-05 [1] CRAN (R 3.6.0) ## glue 1.3.2 2020-03-12 [1] CRAN (R 3.6.0) ## gtable 0.3.0 2019-03-25 [1] CRAN (R 3.6.0) ## HDF5Array 1.14.3 2020-02-29 [1] Bioconductor ## hms 0.5.3 2020-01-08 [1] CRAN (R 3.6.0) ## htmltools 0.4.0 2019-10-04 [1] CRAN (R 3.6.0) ## httpuv 1.5.2 2019-09-11 [1] CRAN (R 3.6.0) ## httr 1.4.1 2019-08-05 [1] CRAN (R 3.6.0) ## interactiveDisplayBase 1.24.0 2019-10-29 [1] Bioconductor ## IRanges * 2.20.2 2020-01-13 [1] Bioconductor ## knitr 1.28 2020-02-06 [1] CRAN (R 3.6.0) ## later 1.0.0 2019-10-04 [1] CRAN (R 3.6.0) ## lattice 0.20-40 2020-02-19 [1] CRAN (R 3.6.0) ## lazyeval 0.2.2 2019-03-15 [1] CRAN (R 3.6.0) ## lifecycle 0.2.0 2020-03-06 [1] CRAN (R 3.6.0) ## limma 3.42.2 2020-02-03 [1] Bioconductor ## locfit 1.5-9.1 2013-04-20 [1] CRAN (R 3.6.0) ## magrittr 1.5 2014-11-22 [1] CRAN (R 3.6.0) ## Matrix 1.2-18 2019-11-27 [1] CRAN (R 3.6.3) ## matrixStats * 0.56.0 2020-03-13 [1] CRAN (R 3.6.0) ## mclust 5.4.5 2019-07-08 [1] CRAN (R 3.6.0) ## memoise 1.1.0 2017-04-21 [1] CRAN (R 3.6.0) ## mime 0.9 2020-02-04 [1] CRAN (R 3.6.0) ## munsell 0.5.0 2018-06-12 [1] CRAN (R 3.6.0) ## openssl 1.4.1 2019-07-18 [1] CRAN (R 3.6.0) ## org.Hs.eg.db 3.10.0 2019-10-31 [1] Bioconductor ## org.Mm.eg.db 3.10.0 2020-03-23 [1] Bioconductor ## pillar 1.4.3 2019-12-20 [1] CRAN (R 3.6.0) ## pkgconfig 2.0.3 2019-09-22 [1] CRAN (R 3.6.1) ## plyr 1.8.6 2020-03-03 [1] CRAN (R 3.6.2) ## prettyunits 1.1.1 2020-01-24 [1] CRAN (R 3.6.2) ## progress 1.2.2 2019-05-16 [1] CRAN (R 3.6.0) ## promises 1.1.0 2019-10-04 [1] CRAN (R 3.6.0) ## ProtGenerics 1.18.0 2019-10-29 [1] Bioconductor ## purrr 0.3.3 2019-10-18 [1] CRAN (R 3.6.0) ## R.methodsS3 1.8.0 2020-02-14 [1] CRAN (R 3.6.0) ## R.oo 1.23.0 2019-11-03 [1] CRAN (R 3.6.0) ## R.utils 2.9.2 2019-12-08 [1] CRAN (R 3.6.1) ## R6 2.4.1 2019-11-12 [1] CRAN (R 3.6.1) ## rappdirs 0.3.1 2016-03-28 [1] CRAN (R 3.6.0) ## RColorBrewer 1.1-2 2014-12-07 [1] CRAN (R 3.6.0) ## Rcpp 1.0.4 2020-03-17 [1] CRAN (R 3.6.0) ## RCurl 1.98-1.1 2020-01-19 [1] CRAN (R 3.6.0) ## reshape 0.8.8 2018-10-23 [1] CRAN (R 3.6.0) ## rhdf5 2.30.1 2019-11-26 [1] Bioconductor ## Rhdf5lib 1.8.0 2019-10-29 [1] Bioconductor ## Rhtslib 1.18.1 2020-01-29 [1] Bioconductor ## rlang 0.4.5 2020-03-01 [1] CRAN (R 3.6.0) ## rmarkdown 2.1 2020-01-20 [1] CRAN (R 3.6.0) ## robustbase 0.93-5 2019-05-12 [1] CRAN (R 3.6.0) ## Rsamtools 2.2.3 2020-02-23 [1] Bioconductor ## RSQLite 2.2.0 2020-01-07 [1] CRAN (R 3.6.0) ## rstudioapi 0.11 2020-02-07 [1] CRAN (R 3.6.0) ## rtracklayer 1.46.0 2019-10-29 [1] Bioconductor ## S4Vectors * 0.24.3 2020-01-18 [1] Bioconductor ## scales 1.1.0 2019-11-18 [1] CRAN (R 3.6.1) ## scPipe * 1.8.0 2019-10-29 [1] Bioconductor ## scRNAseq * 2.0.2 2019-11-12 [1] Bioconductor ## sessioninfo 1.1.1 2018-11-05 [1] CRAN (R 3.6.0) ## shiny 1.4.0.2 2020-03-13 [1] CRAN (R 3.6.0) ## SingleCellExperiment * 1.8.0 2019-10-29 [1] Bioconductor ## stringi 1.4.6 2020-02-17 [1] CRAN (R 3.6.0) ## stringr 1.4.0 2019-02-10 [1] CRAN (R 3.6.0) ## SummarizedExperiment * 1.16.1 2019-12-19 [1] Bioconductor ## tibble 2.1.3 2019-06-06 [1] CRAN (R 3.6.0) ## tidyselect 1.0.0 2020-01-27 [1] CRAN (R 3.6.0) ## vctrs 0.2.4 2020-03-10 [1] CRAN (R 3.6.0) ## withr 2.1.2 2018-03-15 [1] CRAN (R 3.6.0) ## xaringan 0.15 2020-03-04 [1] CRAN (R 3.6.3) ## xaringanthemer * 0.2.0 2020-03-22 [1] Github (gadenbuie/xaringanthemer@460f441) ## xfun 0.12 2020-01-13 [1] CRAN (R 3.6.0) ## XML 3.99-0.3 2020-01-20 [1] CRAN (R 3.6.0) ## xtable 1.8-4 2019-04-21 [1] CRAN (R 3.6.0) ## XVector 0.26.0 2019-10-29 [1] Bioconductor ## yaml 2.2.1 2020-02-01 [1] CRAN (R 3.6.0) ## zlibbioc 1.32.0 2019-10-29 [1] Bioconductor ## ## [1] /Library/Frameworks/R.framework/Versions/3.6/Resources/library ``` ]]