class: center, middle, inverse, title-slide #
Quality Control
## Analyzing
scRNA-seq
data with
Bioconductor
for
LCG-EJ-UNAM
March 2020 ###
Leonardo Collado-Torres
### 2020-03-23 --- class: inverse .center[ <a href="https://bioconductor.org/"><img src="https://osca.bioconductor.org/cover.png" style="width: 30%"/></a> <a rel="license" href="http://creativecommons.org/licenses/by-nc-sa/4.0/"><img alt="Creative Commons License" style="border-width:0" src="https://i.creativecommons.org/l/by-nc-sa/4.0/88x31.png" /></a><br />This work is licensed under a <a rel="license" href="http://creativecommons.org/licenses/by-nc-sa/4.0/">Creative Commons Attribution-NonCommercial-ShareAlike 4.0 International License</a>. <a href='https://clustrmaps.com/site/1b5pl' title='Visit tracker'><img src='//clustrmaps.com/map_v2.png?cl=ffffff&w=150&t=n&d=tq5q8216epOrQBSllNIKhXOHUHi-i38brzUURkQEiXw'/></a> ] .footnote[ Download the materials for this course with `usethis::use_course('lcolladotor/osca_LIIGH_UNAM_2020')` or view online at [**lcolladotor.github.io/osca_LIIGH_UNAM_2020**](http://lcolladotor.github.io/osca_LIIGH_UNAM_2020).] <style type="text/css"> /* From https://github.com/yihui/xaringan/issues/147 */ .scroll-output { height: 80%; overflow-y: scroll; } /* https://stackoverflow.com/questions/50919104/horizontally-scrollable-output-on-xaringan-slides */ pre { max-width: 100%; overflow-x: scroll; } /* From https://github.com/yihui/xaringan/wiki/Font-Size */ .tiny{ font-size: 40% } /* From https://github.com/yihui/xaringan/wiki/Title-slide */ .title-slide { background-image: url(https://raw.githubusercontent.com/Bioconductor/OrchestratingSingleCellAnalysis/master/images/Workflow.png); background-size: 33%; background-position: 0% 100% } </style> --- # Slides by Peter Hickey View them [here](https://docs.google.com/presentation/d/1pIiA7fZd1GBxaKQpzT2sPn7C6fIZxEJ8NI4p3UtoIOo/edit#slide=id.p) --- # Code and output .scroll-output[ ```r ## Data library('scRNAseq') sce.416b <- LunSpikeInData(which = "416b") ``` ``` ## snapshotDate(): 2019-10-22 ``` ``` ## see ?scRNAseq and browseVignettes('scRNAseq') for documentation ``` ``` ## loading from cache ``` ``` ## see ?scRNAseq and browseVignettes('scRNAseq') for documentation ``` ``` ## loading from cache ``` ``` ## see ?scRNAseq and browseVignettes('scRNAseq') for documentation ``` ``` ## loading from cache ``` ```r sce.416b$block <- factor(sce.416b$block) # Download the relevant Ensembl annotation database # using AnnotationHub resources library('AnnotationHub') ah <- AnnotationHub() ``` ``` ## snapshotDate(): 2019-10-29 ``` ```r query(ah, c("Mus musculus", "Ensembl", "v97")) ``` ``` ## AnnotationHub with 1 record ## # snapshotDate(): 2019-10-29 ## # names(): AH73905 ## # $dataprovider: Ensembl ## # $species: Mus musculus ## # $rdataclass: EnsDb ## # $rdatadateadded: 2019-05-02 ## # $title: Ensembl 97 EnsDb for Mus musculus ## # $description: Gene and protein annotations for Mus musculus based on Ensem... ## # $taxonomyid: 10090 ## # $genome: GRCm38 ## # $sourcetype: ensembl ## # $sourceurl: http://www.ensembl.org ## # $sourcesize: NA ## # $tags: c("97", "AHEnsDbs", "Annotation", "EnsDb", "Ensembl", "Gene", ## # "Protein", "Transcript") ## # retrieve record with 'object[["AH73905"]]' ``` ```r # Annotate each gene with its chromosome location ens.mm.v97 <- ah[["AH73905"]] ``` ``` ## loading from cache ``` ```r location <- mapIds( ens.mm.v97, keys = rownames(sce.416b), keytype = "GENEID", column = "SEQNAME" ) ``` ``` ## Warning: Unable to map 563 of 46604 requested IDs. ``` ```r # Identify the mitochondrial genes is.mito <- which(location == "MT") library('scater') sce.416b <- addPerCellQC(sce.416b, subsets = list(Mito = is.mito)) ``` ] --- # Questions -- * What changed in our `sce` object after `addPerCellQC`? -- * Plot boxplots of the number of detected genes by the sample block. ??? * We now have more information at `colData(sce.416b)` * `with(colData(sce.416b), boxplot(detected ~ block))` --- # QC metrics plots .scroll-output[ ```r plotColData(sce.416b, x = "block", y = "detected") ``` ``` ## Warning: partial match of 'val' to 'value' ## Warning: partial match of 'val' to 'value' ``` 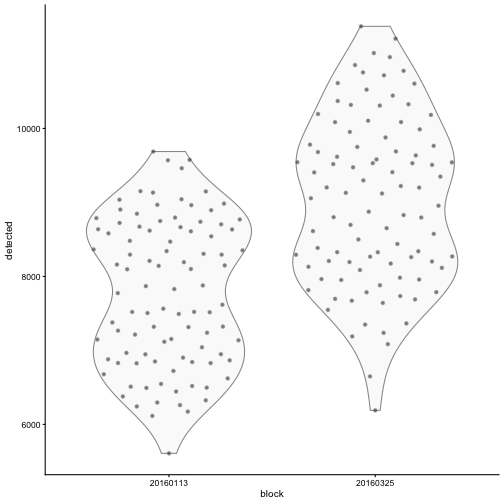<!-- --> ```r plotColData(sce.416b, x = "block", y = "detected") + scale_y_log10() ``` ``` ## Warning: partial match of 'val' to 'value' ## Warning: partial match of 'val' to 'value' ``` 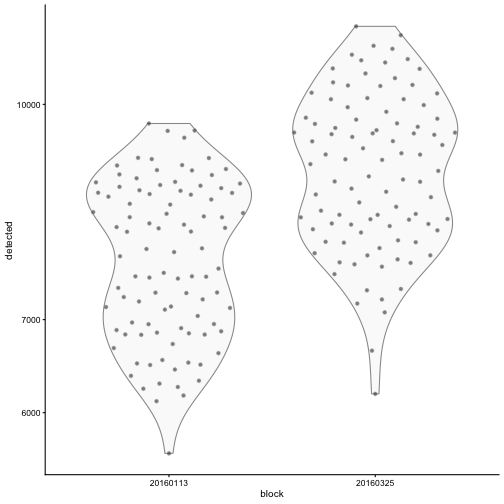<!-- --> ```r plotColData(sce.416b, x = "block", y = "detected", other_fields = "phenotype") + scale_y_log10() + facet_wrap( ~ phenotype) ``` ``` ## Warning: partial match of 'val' to 'value' ## Warning: partial match of 'val' to 'value' ``` 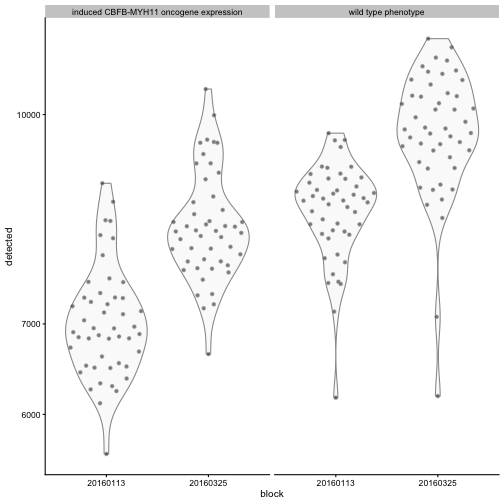<!-- --> ] --- .scroll-output[ ```r # Example thresholds qc.lib <- sce.416b$sum < 100000 qc.nexprs <- sce.416b$detected < 5000 qc.spike <- sce.416b$altexps_ERCC_percent > 10 qc.mito <- sce.416b$subsets_Mito_percent > 10 discard <- qc.lib | qc.nexprs | qc.spike | qc.mito # Summarize the number of cells removed for each reason DataFrame( LibSize = sum(qc.lib), NExprs = sum(qc.nexprs), SpikeProp = sum(qc.spike), MitoProp = sum(qc.mito), Total = sum(discard) ) ``` ``` ## DataFrame with 1 row and 5 columns ## LibSize NExprs SpikeProp MitoProp Total ## <integer> <integer> <integer> <integer> <integer> ## 1 3 0 19 14 33 ``` ```r qc.lib2 <- isOutlier(sce.416b$sum, log = TRUE, type = "lower") qc.nexprs2 <- isOutlier(sce.416b$detected, log = TRUE, type = "lower") qc.spike2 <- isOutlier(sce.416b$altexps_ERCC_percent, type = "higher") qc.mito2 <- isOutlier(sce.416b$subsets_Mito_percent, type = "higher") discard2 <- qc.lib2 | qc.nexprs2 | qc.spike2 | qc.mito2 # Extract the thresholds attr(qc.lib2, "thresholds") ``` ``` ## lower higher ## 434082.9 Inf ``` ```r attr(qc.nexprs2, "thresholds") ``` ``` ## lower higher ## 5231.468 Inf ``` ```r # Summarize the number of cells removed for each reason. DataFrame( LibSize = sum(qc.lib2), NExprs = sum(qc.nexprs2), SpikeProp = sum(qc.spike2), MitoProp = sum(qc.mito2), Total = sum(discard2) ) ``` ``` ## DataFrame with 1 row and 5 columns ## LibSize NExprs SpikeProp MitoProp Total ## <integer> <integer> <integer> <integer> <integer> ## 1 4 0 1 2 6 ``` ```r ## More checks plotColData(sce.416b, x = "block", y = "detected", other_fields = "phenotype") + scale_y_log10() + facet_wrap( ~ phenotype) ``` ``` ## Warning: partial match of 'val' to 'value' ## Warning: partial match of 'val' to 'value' ``` 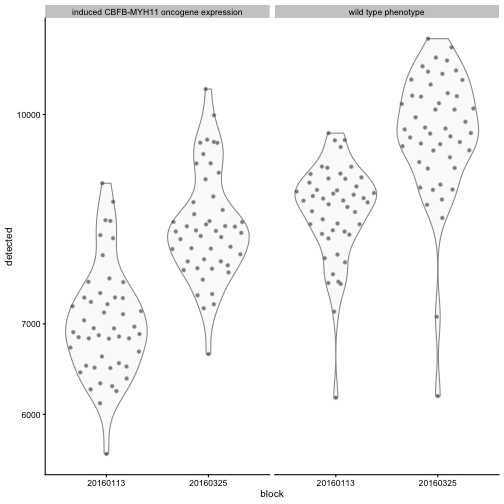<!-- --> ```r batch <- paste0(sce.416b$phenotype, "-", sce.416b$block) qc.lib3 <- isOutlier(sce.416b$sum, log = TRUE, type = "lower", batch = batch) qc.nexprs3 <- isOutlier(sce.416b$detected, log = TRUE, type = "lower", batch = batch) qc.spike3 <- isOutlier(sce.416b$altexps_ERCC_percent, type = "higher", batch = batch) qc.mito3 <- isOutlier(sce.416b$subsets_Mito_percent, type = "higher", batch = batch) discard3 <- qc.lib3 | qc.nexprs3 | qc.spike3 | qc.mito3 # Extract the thresholds attr(qc.lib3, "thresholds") ``` ``` ## induced CBFB-MYH11 oncogene expression-20160113 ## lower 461073.1 ## higher Inf ## induced CBFB-MYH11 oncogene expression-20160325 ## lower 399133.7 ## higher Inf ## wild type phenotype-20160113 wild type phenotype-20160325 ## lower 599794.9 370316.5 ## higher Inf Inf ``` ```r attr(qc.nexprs3, "thresholds") ``` ``` ## induced CBFB-MYH11 oncogene expression-20160113 ## lower 5399.24 ## higher Inf ## induced CBFB-MYH11 oncogene expression-20160325 ## lower 6519.74 ## higher Inf ## wild type phenotype-20160113 wild type phenotype-20160325 ## lower 7215.887 7586.402 ## higher Inf Inf ``` ```r # Summarize the number of cells removed for each reason DataFrame( LibSize = sum(qc.lib3), NExprs = sum(qc.nexprs3), SpikeProp = sum(qc.spike3), MitoProp = sum(qc.mito3), Total = sum(discard3) ) ``` ``` ## DataFrame with 1 row and 5 columns ## LibSize NExprs SpikeProp MitoProp Total ## <integer> <integer> <integer> <integer> <integer> ## 1 5 4 6 2 9 ``` ] --- # Questions -- * Was `qc.lib` necessary for creating `discord`? -- * Which filter discarded more cells? `discard` or `discard2`? -- * By considering the sample batch, did we discard more cells using automatic threshold detection? ??? * Yes from `table(qc.lib , qc.spike)` and `table(qc.lib , qc.mito)` * `discard` from `table(discard, discard2)` * Yes from `table(discard, discard2, discard3)` --- .scroll-output[ ```r sce.grun <- GrunPancreasData() ``` ``` ## snapshotDate(): 2019-10-22 ``` ``` ## see ?scRNAseq and browseVignettes('scRNAseq') for documentation ``` ``` ## loading from cache ``` ```r sce.grun <- addPerCellQC(sce.grun) plotColData(sce.grun, x = "donor", y = "altexps_ERCC_percent") ``` ``` ## Warning: partial match of 'val' to 'value' ## Warning: partial match of 'val' to 'value' ``` ``` ## Warning: Removed 10 rows containing non-finite values (stat_ydensity). ``` ``` ## Warning: Removed 10 rows containing missing values (position_quasirandom). ``` 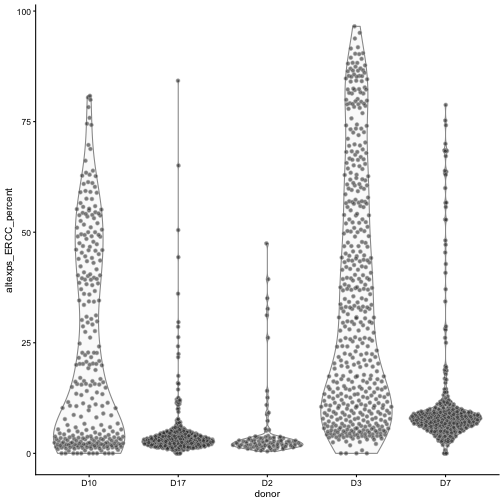<!-- --> ```r discard.ercc <- isOutlier(sce.grun$altexps_ERCC_percent, type = "higher", batch = sce.grun$donor) ``` ``` ## Warning in isOutlier(sce.grun$altexps_ERCC_percent, type = "higher", batch = ## sce.grun$donor): missing values ignored during outlier detection ``` ```r discard.ercc2 <- isOutlier( sce.grun$altexps_ERCC_percent, type = "higher", batch = sce.grun$donor, subset = sce.grun$donor %in% c("D17", "D2", "D7") ) ``` ``` ## Warning in isOutlier(sce.grun$altexps_ERCC_percent, type = "higher", batch = ## sce.grun$donor, : missing values ignored during outlier detection ``` ```r plotColData( sce.grun, x = "donor", y = "altexps_ERCC_percent", colour_by = data.frame(discard = discard.ercc) ) ``` ``` ## Warning: partial match of 'val' to 'value' ``` ``` ## Warning: partial match of 'val' to 'value' ``` ``` ## Warning: Removed 10 rows containing non-finite values (stat_ydensity). ``` ``` ## Warning: Removed 10 rows containing missing values (position_quasirandom). ``` 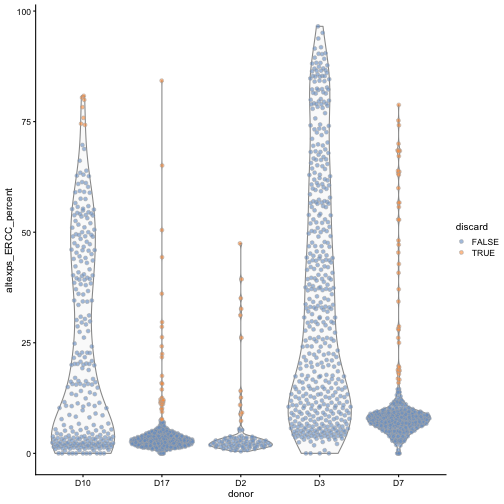<!-- --> ```r plotColData( sce.grun, x = "donor", y = "altexps_ERCC_percent", colour_by = data.frame(discard = discard.ercc2) ) ``` ``` ## Warning: partial match of 'val' to 'value' ``` ``` ## Warning: partial match of 'val' to 'value' ``` ``` ## Warning: Removed 10 rows containing non-finite values (stat_ydensity). ``` ``` ## Warning: Removed 10 rows containing missing values (position_quasirandom). ``` 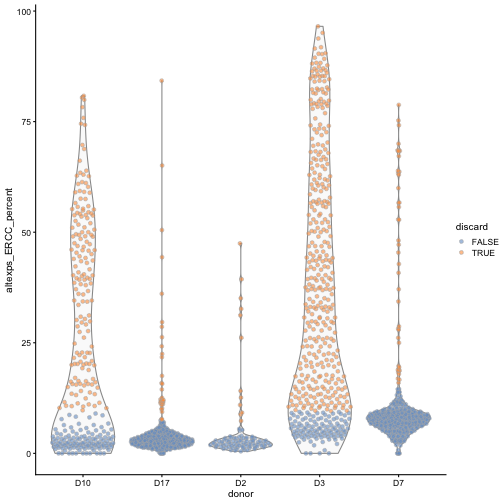<!-- --> ```r # Add info about which cells are outliers sce.416b$discard <- discard2 # Look at this plot for each QC metric plotColData( sce.416b, x = "block", y = "sum", colour_by = "discard", other_fields = "phenotype" ) + facet_wrap( ~ phenotype) + scale_y_log10() ``` ``` ## Warning: partial match of 'val' to 'value' ``` ``` ## Warning: partial match of 'val' to 'value' ``` 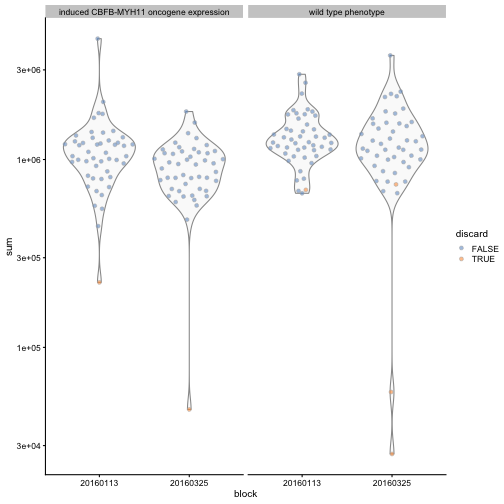<!-- --> ```r # Another useful diagnostic plot plotColData( sce.416b, x = "sum", y = "subsets_Mito_percent", colour_by = "discard", other_fields = c("block", "phenotype") ) + facet_grid(block ~ phenotype) ``` ``` ## Warning: partial match of 'val' to 'value' ## Warning: partial match of 'val' to 'value' ``` 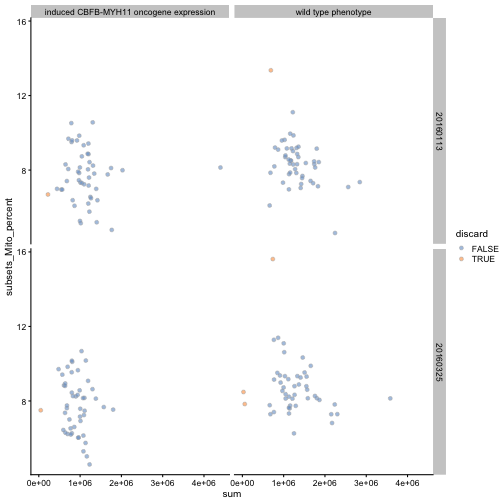<!-- --> ] --- .scroll-output[ ```r library('BiocFileCache') bfc <- BiocFileCache() raw.path <- bfcrpath( bfc, file.path( "http://cf.10xgenomics.com/samples", "cell-exp/2.1.0/pbmc4k/pbmc4k_raw_gene_bc_matrices.tar.gz" ) ) untar(raw.path, exdir = file.path(tempdir(), "pbmc4k")) library('DropletUtils') library('Matrix') ``` ``` ## ## Attaching package: 'Matrix' ``` ``` ## The following object is masked from 'package:S4Vectors': ## ## expand ``` ```r fname <- file.path(tempdir(), "pbmc4k/raw_gene_bc_matrices/GRCh38") sce.pbmc <- read10xCounts(fname, col.names = TRUE) bcrank <- barcodeRanks(counts(sce.pbmc)) # Only showing unique points for plotting speed. uniq <- !duplicated(bcrank$rank) plot( bcrank$rank[uniq], bcrank$total[uniq], log = "xy", xlab = "Rank", ylab = "Total UMI count", cex.lab = 1.2 ) ``` ``` ## Warning in xy.coords(x, y, xlabel, ylabel, log): 1 y value <= 0 omitted from ## logarithmic plot ``` ```r abline(h = metadata(bcrank)$inflection, col = "darkgreen", lty = 2) abline(h = metadata(bcrank)$knee, col = "dodgerblue", lty = 2) legend( "bottomleft", legend = c("Inflection", "Knee"), col = c("darkgreen", "dodgerblue"), lty = 2, cex = 1.2 ) ``` 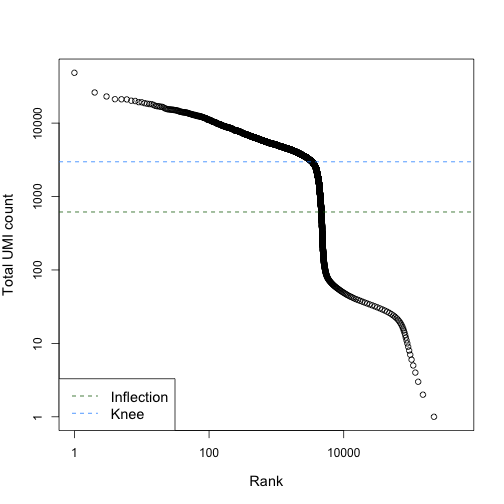<!-- --> ```r set.seed(100) e.out <- emptyDrops(counts(sce.pbmc)) # See ?emptyDrops for an explanation of why there are NA # values. summary(e.out$FDR <= 0.001) ``` ``` ## Mode FALSE TRUE NA's ## logical 1056 4233 731991 ``` ```r set.seed(100) limit <- 100 all.out <- emptyDrops(counts(sce.pbmc), lower = limit, test.ambient = TRUE) # Ideally, this histogram should look close to uniform. # Large peaks near zero indicate that barcodes with total # counts below 'lower' are not ambient in origin. hist(all.out$PValue[all.out$Total <= limit & all.out$Total > 0], xlab = "P-value", main = "", col = "grey80") ``` 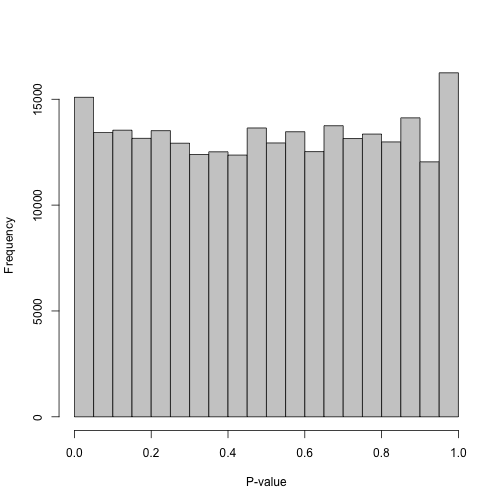<!-- --> ```r sce.pbmc <- sce.pbmc[, which(e.out$FDR <= 0.001)] is.mito <- grep("^MT-", rowData(sce.pbmc)$Symbol) sce.pmbc <- addPerCellQC(sce.pbmc, subsets = list(MT = is.mito)) discard.mito <- isOutlier(sce.pmbc$subsets_MT_percent, type = "higher") plot( sce.pmbc$sum, sce.pmbc$subsets_MT_percent, log = "x", xlab = "Total count", ylab = "Mitochondrial %" ) abline(h = attr(discard.mito, "thresholds")["higher"], col = "red") ``` 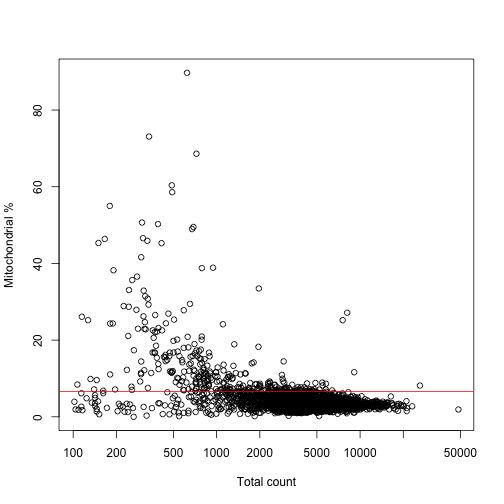<!-- --> ] --- # Exercises -- * Why does `emptyDrops()` return `NA` values? -- * Are the p-values the same for `e.out` and `all.out`? -- * What if you subset to the non-`NA` entries? ??? * Below `lower` they are considered emptry droplets. Only used for multiple correction testing. * No, due to the `NA`s. * Yes: `identical(e.out$PValue[!is.na(e.out$FDR)], all.out$PValue[!is.na(e.out$FDR)])` --- ```r # Removing low-quality cells # Keeping the columns we DON'T want to discard filtered <- sce.416b[,!discard2] # Marking low-quality cells marked <- sce.416b marked$discard <- discard2 ``` -- * Which of these objects is larger? -- * Which one would you prefer to use? ??? * `marked` is larger than `filtered` * I would prefer to use the `marked` one if I have enough memory to do so. --- class: middle .center[ # Thanks! Slides created via the R package [**xaringan**](https://github.com/yihui/xaringan) and themed with [**xaringanthemer**](https://github.com/gadenbuie/xaringanthemer). This course is based on the book [**Orchestrating Single Cell Analysis with Bioconductor**](https://osca.bioconductor.org/) by [Aaron Lun](https://www.linkedin.com/in/aaron-lun-869b5894/), [Robert Amezquita](https://robertamezquita.github.io/), [Stephanie Hicks](https://www.stephaniehicks.com/) and [Raphael Gottardo](http://rglab.org), plus [**WEHI's scRNA-seq course**](https://drive.google.com/drive/folders/1cn5d-Ey7-kkMiex8-74qxvxtCQT6o72h) by [Peter Hickey](https://www.peterhickey.org/). You can find the files for this course at [lcolladotor/osca_LIIGH_UNAM_2020](https://github.com/lcolladotor/osca_LIIGH_UNAM_2020). Instructor: [**Leonardo Collado-Torres**](http://lcolladotor.github.io/). <a href="https://www.libd.org"><img src="img/LIBD_logo.jpg" style="width: 20%" /></a> ] .footnote[ Download the materials for this course with `usethis::use_course('lcolladotor/osca_LIIGH_UNAM_2020')` or view online at [**lcolladotor.github.io/osca_LIIGH_UNAM_2020**](http://lcolladotor.github.io/osca_LIIGH_UNAM_2020).] --- # R session information .scroll-output[ .tiny[ ```r options(width = 120) sessioninfo::session_info() ``` ``` ## ─ Session info ─────────────────────────────────────────────────────────────────────────────────────────────────────── ## setting value ## version R version 3.6.3 (2020-02-29) ## os macOS Catalina 10.15.3 ## system x86_64, darwin15.6.0 ## ui X11 ## language (EN) ## collate en_US.UTF-8 ## ctype en_US.UTF-8 ## tz America/New_York ## date 2020-03-23 ## ## ─ Packages ─────────────────────────────────────────────────────────────────────────────────────────────────────────── ## package * version date lib source ## AnnotationDbi * 1.48.0 2019-10-29 [1] Bioconductor ## AnnotationFilter * 1.10.0 2019-10-29 [1] Bioconductor ## AnnotationHub * 2.18.0 2019-10-29 [1] Bioconductor ## askpass 1.1 2019-01-13 [1] CRAN (R 3.6.0) ## assertthat 0.2.1 2019-03-21 [1] CRAN (R 3.6.0) ## beeswarm 0.2.3 2016-04-25 [1] CRAN (R 3.6.0) ## Biobase * 2.46.0 2019-10-29 [1] Bioconductor ## BiocFileCache * 1.10.2 2019-11-08 [1] Bioconductor ## BiocGenerics * 0.32.0 2019-10-29 [1] Bioconductor ## BiocManager 1.30.10 2019-11-16 [1] CRAN (R 3.6.1) ## BiocNeighbors 1.4.2 2020-02-29 [1] Bioconductor ## BiocParallel * 1.20.1 2019-12-21 [1] Bioconductor ## BiocSingular 1.2.2 2020-02-14 [1] Bioconductor ## BiocVersion 3.10.1 2019-06-06 [1] Bioconductor ## biomaRt 2.42.0 2019-10-29 [1] Bioconductor ## Biostrings 2.54.0 2019-10-29 [1] Bioconductor ## bit 1.1-15.2 2020-02-10 [1] CRAN (R 3.6.0) ## bit64 0.9-7 2017-05-08 [1] CRAN (R 3.6.0) ## bitops 1.0-6 2013-08-17 [1] CRAN (R 3.6.0) ## blob 1.2.1 2020-01-20 [1] CRAN (R 3.6.0) ## cli 2.0.2 2020-02-28 [1] CRAN (R 3.6.0) ## colorout * 1.2-1 2019-05-07 [1] Github (jalvesaq/colorout@7ea9440) ## colorspace 1.4-1 2019-03-18 [1] CRAN (R 3.6.0) ## crayon 1.3.4 2017-09-16 [1] CRAN (R 3.6.0) ## curl 4.3 2019-12-02 [1] CRAN (R 3.6.0) ## DBI 1.1.0 2019-12-15 [1] CRAN (R 3.6.0) ## dbplyr * 1.4.2 2019-06-17 [1] CRAN (R 3.6.0) ## DelayedArray * 0.12.2 2020-01-06 [1] Bioconductor ## DelayedMatrixStats 1.8.0 2019-10-29 [1] Bioconductor ## digest 0.6.25 2020-02-23 [1] CRAN (R 3.6.0) ## dplyr 0.8.5 2020-03-07 [1] CRAN (R 3.6.0) ## dqrng 0.2.1 2019-05-17 [1] CRAN (R 3.6.0) ## DropletUtils * 1.6.1 2019-10-30 [1] Bioconductor ## edgeR 3.28.1 2020-02-26 [1] Bioconductor ## ensembldb * 2.10.2 2019-11-20 [1] Bioconductor ## evaluate 0.14 2019-05-28 [1] CRAN (R 3.6.0) ## ExperimentHub 1.12.0 2019-10-29 [1] Bioconductor ## fansi 0.4.1 2020-01-08 [1] CRAN (R 3.6.0) ## fastmap 1.0.1 2019-10-08 [1] CRAN (R 3.6.0) ## GenomeInfoDb * 1.22.0 2019-10-29 [1] Bioconductor ## GenomeInfoDbData 1.2.2 2019-10-31 [1] Bioconductor ## GenomicAlignments 1.22.1 2019-11-12 [1] Bioconductor ## GenomicFeatures * 1.38.2 2020-02-15 [1] Bioconductor ## GenomicRanges * 1.38.0 2019-10-29 [1] Bioconductor ## ggbeeswarm 0.6.0 2017-08-07 [1] CRAN (R 3.6.0) ## ggplot2 * 3.3.0 2020-03-05 [1] CRAN (R 3.6.0) ## glue 1.3.2 2020-03-12 [1] CRAN (R 3.6.0) ## gridExtra 2.3 2017-09-09 [1] CRAN (R 3.6.0) ## gtable 0.3.0 2019-03-25 [1] CRAN (R 3.6.0) ## HDF5Array 1.14.3 2020-02-29 [1] Bioconductor ## hms 0.5.3 2020-01-08 [1] CRAN (R 3.6.0) ## htmltools 0.4.0 2019-10-04 [1] CRAN (R 3.6.0) ## httpuv 1.5.2 2019-09-11 [1] CRAN (R 3.6.0) ## httr 1.4.1 2019-08-05 [1] CRAN (R 3.6.0) ## interactiveDisplayBase 1.24.0 2019-10-29 [1] Bioconductor ## IRanges * 2.20.2 2020-01-13 [1] Bioconductor ## irlba 2.3.3 2019-02-05 [1] CRAN (R 3.6.0) ## knitr 1.28 2020-02-06 [1] CRAN (R 3.6.0) ## later 1.0.0 2019-10-04 [1] CRAN (R 3.6.0) ## lattice 0.20-40 2020-02-19 [1] CRAN (R 3.6.0) ## lazyeval 0.2.2 2019-03-15 [1] CRAN (R 3.6.0) ## lifecycle 0.2.0 2020-03-06 [1] CRAN (R 3.6.0) ## limma 3.42.2 2020-02-03 [1] Bioconductor ## locfit 1.5-9.1 2013-04-20 [1] CRAN (R 3.6.0) ## magrittr 1.5 2014-11-22 [1] CRAN (R 3.6.0) ## Matrix * 1.2-18 2019-11-27 [1] CRAN (R 3.6.3) ## matrixStats * 0.56.0 2020-03-13 [1] CRAN (R 3.6.0) ## memoise 1.1.0 2017-04-21 [1] CRAN (R 3.6.0) ## mime 0.9 2020-02-04 [1] CRAN (R 3.6.0) ## munsell 0.5.0 2018-06-12 [1] CRAN (R 3.6.0) ## openssl 1.4.1 2019-07-18 [1] CRAN (R 3.6.0) ## pillar 1.4.3 2019-12-20 [1] CRAN (R 3.6.0) ## pkgconfig 2.0.3 2019-09-22 [1] CRAN (R 3.6.1) ## prettyunits 1.1.1 2020-01-24 [1] CRAN (R 3.6.2) ## progress 1.2.2 2019-05-16 [1] CRAN (R 3.6.0) ## promises 1.1.0 2019-10-04 [1] CRAN (R 3.6.0) ## ProtGenerics 1.18.0 2019-10-29 [1] Bioconductor ## purrr 0.3.3 2019-10-18 [1] CRAN (R 3.6.0) ## R.methodsS3 1.8.0 2020-02-14 [1] CRAN (R 3.6.0) ## R.oo 1.23.0 2019-11-03 [1] CRAN (R 3.6.0) ## R.utils 2.9.2 2019-12-08 [1] CRAN (R 3.6.1) ## R6 2.4.1 2019-11-12 [1] CRAN (R 3.6.1) ## rappdirs 0.3.1 2016-03-28 [1] CRAN (R 3.6.0) ## Rcpp 1.0.4 2020-03-17 [1] CRAN (R 3.6.0) ## RCurl 1.98-1.1 2020-01-19 [1] CRAN (R 3.6.0) ## rhdf5 2.30.1 2019-11-26 [1] Bioconductor ## Rhdf5lib 1.8.0 2019-10-29 [1] Bioconductor ## rlang 0.4.5 2020-03-01 [1] CRAN (R 3.6.0) ## rmarkdown 2.1 2020-01-20 [1] CRAN (R 3.6.0) ## Rsamtools 2.2.3 2020-02-23 [1] Bioconductor ## RSQLite 2.2.0 2020-01-07 [1] CRAN (R 3.6.0) ## rstudioapi 0.11 2020-02-07 [1] CRAN (R 3.6.0) ## rsvd 1.0.3 2020-02-17 [1] CRAN (R 3.6.0) ## rtracklayer 1.46.0 2019-10-29 [1] Bioconductor ## S4Vectors * 0.24.3 2020-01-18 [1] Bioconductor ## scales 1.1.0 2019-11-18 [1] CRAN (R 3.6.1) ## scater * 1.14.6 2019-12-16 [1] Bioconductor ## scRNAseq * 2.0.2 2019-11-12 [1] Bioconductor ## sessioninfo 1.1.1 2018-11-05 [1] CRAN (R 3.6.0) ## shiny 1.4.0.2 2020-03-13 [1] CRAN (R 3.6.0) ## SingleCellExperiment * 1.8.0 2019-10-29 [1] Bioconductor ## stringi 1.4.6 2020-02-17 [1] CRAN (R 3.6.0) ## stringr 1.4.0 2019-02-10 [1] CRAN (R 3.6.0) ## SummarizedExperiment * 1.16.1 2019-12-19 [1] Bioconductor ## tibble 2.1.3 2019-06-06 [1] CRAN (R 3.6.0) ## tidyselect 1.0.0 2020-01-27 [1] CRAN (R 3.6.0) ## vctrs 0.2.4 2020-03-10 [1] CRAN (R 3.6.0) ## vipor 0.4.5 2017-03-22 [1] CRAN (R 3.6.0) ## viridis 0.5.1 2018-03-29 [1] CRAN (R 3.6.0) ## viridisLite 0.3.0 2018-02-01 [1] CRAN (R 3.6.0) ## withr 2.1.2 2018-03-15 [1] CRAN (R 3.6.0) ## xaringan 0.15 2020-03-04 [1] CRAN (R 3.6.3) ## xaringanthemer * 0.2.0 2020-03-22 [1] Github (gadenbuie/xaringanthemer@460f441) ## xfun 0.12 2020-01-13 [1] CRAN (R 3.6.0) ## XML 3.99-0.3 2020-01-20 [1] CRAN (R 3.6.0) ## xtable 1.8-4 2019-04-21 [1] CRAN (R 3.6.0) ## XVector 0.26.0 2019-10-29 [1] Bioconductor ## yaml 2.2.1 2020-02-01 [1] CRAN (R 3.6.0) ## zlibbioc 1.32.0 2019-10-29 [1] Bioconductor ## ## [1] /Library/Frameworks/R.framework/Versions/3.6/Resources/library ``` ]]